科學(xué)家的主要作用是從數(shù)據(jù)中提取基礎(chǔ)知識(shí)。材料科學(xué)中機(jī)器學(xué)習(xí)的目標(biāo)是通過自動(dòng)識(shí)別關(guān)鍵數(shù)據(jù)之間的關(guān)系來獲得科學(xué)知識(shí)的深入理解,從而加速基礎(chǔ)科學(xué)研究。但如何自動(dòng)識(shí)別關(guān)鍵數(shù)據(jù)之間的關(guān)系尚需深入研究。
來自美國加州理工學(xué)院的John M. Gregoire領(lǐng)導(dǎo)的團(tuán)隊(duì)證明,通過分析訓(xùn)練的神經(jīng)網(wǎng)絡(luò)模型本身,可以加速上述知識(shí)獲取過程。以BiVO4基光陽極為例,他們使用訓(xùn)練的卷積神經(jīng)網(wǎng)絡(luò)預(yù)測了該體系的光電化學(xué)性能。他們利用高通量實(shí)驗(yàn)獲得的1379個(gè)光陽極樣品的組成和拉曼光譜來訓(xùn)練神經(jīng)網(wǎng)絡(luò)模型。該模型的梯度能有效地可視化材料參數(shù)空間中特定區(qū)域的數(shù)據(jù)規(guī)律,以及整個(gè)數(shù)據(jù)集的數(shù)據(jù)規(guī)律。梯度自動(dòng)分析為材料研究提供了指導(dǎo),包括如何超越現(xiàn)有數(shù)據(jù)集的限制,以進(jìn)一步提高材料性能。這種解釋機(jī)器學(xué)習(xí)模型的方法加速了人們對材料科學(xué)的認(rèn)識(shí),并揭示了科學(xué)發(fā)現(xiàn)的自動(dòng)化途徑。
該文近期發(fā)表于npj Computational Materials5:34 (2019),英文標(biāo)題與摘要如下,點(diǎn)擊左下角“閱讀原文”可以自由獲取論文PDF。
Analyzing machine learning models to accelerate generation of fundamental materials insights
Mitsutaro Umehara, Helge S. Stein, Dan Guevarra, Paul F. Newhouse, David A. Boyd & John M. Gregoire
Machine learning for materials science envisions the acceleration of basic science research through automated identification of key data relationships to augment human interpretation and gain scientific understanding. A primary role of scientists is extraction of fundamental knowledge from data, and we demonstrate that this extraction can be accelerated using neural networks via analysis of the trained data model itself rather than its application as a prediction tool. Convolutional neural networks excel at modeling complex data relationships in multi-dimensional parameter spaces, such as that mapped by a combinatorial materials science experiment. Measuring a performance metric in a given materials space provides direct information about (locally) optimal materials but not the underlying materials science that gives rise to the variation in performance. By building a model that predicts performance (in this case photoelectrochemical power generation of a solar fuels photoanode) from materials parameters (in this case composition and Raman signal), subsequent analysis of gradients in the trained model reveals key data relationships that are not readily identified by human inspection or traditional statistical analyses. Human interpretation of these key relationships produces the desired fundamental understanding, demonstrating a framework in which machine learning accelerates data interpretation by leveraging the expertize of the human scientist. We also demonstrate the use of neural network gradient analysis to automate prediction of the directions in parameter space, such as the addition of specific alloying elements, that may increase performance by moving beyond the confines of existing data.
-
機(jī)器學(xué)習(xí)
+關(guān)注
關(guān)注
66文章
8418瀏覽量
132654 -
機(jī)器學(xué)習(xí)模型
+關(guān)注
關(guān)注
0文章
9瀏覽量
2582
原文標(biāo)題:npj: 分析機(jī)器學(xué)習(xí)模型-加速材料的基礎(chǔ)認(rèn)識(shí)
文章出處:【微信號:zhishexueshuquan,微信公眾號:知社學(xué)術(shù)圈】歡迎添加關(guān)注!文章轉(zhuǎn)載請注明出處。
發(fā)布評論請先 登錄
相關(guān)推薦
機(jī)器學(xué)習(xí)模型可解釋性的結(jié)果分析
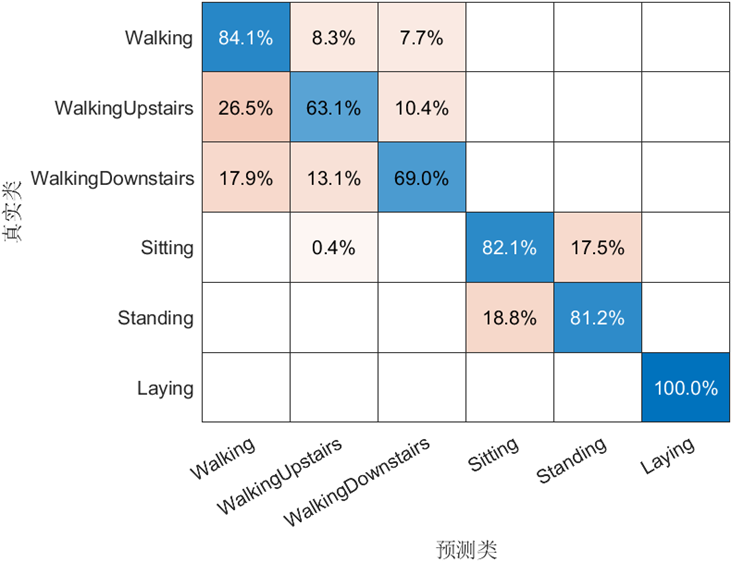
機(jī)器學(xué)習(xí)模型評估指標(biāo)
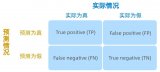
如何使用TensorFlow構(gòu)建機(jī)器學(xué)習(xí)模型
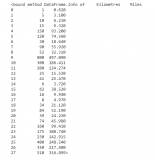
【下載】《機(jī)器學(xué)習(xí)》+《機(jī)器學(xué)習(xí)實(shí)戰(zhàn)》
什么是機(jī)器學(xué)習(xí)? 機(jī)器學(xué)習(xí)基礎(chǔ)入門
部署基于嵌入的機(jī)器學(xué)習(xí)模型
如何才能正確的構(gòu)建機(jī)器學(xué)習(xí)的模型
詳談機(jī)器學(xué)習(xí)模型算法的質(zhì)量保障方案
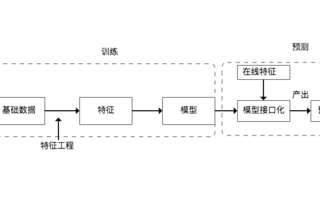
六個(gè)構(gòu)建機(jī)器學(xué)習(xí)模型需避免的錯(cuò)誤
機(jī)器學(xué)習(xí)模型在功耗分析攻擊中的研究
使用TensorBoard的機(jī)器學(xué)習(xí)模型分析
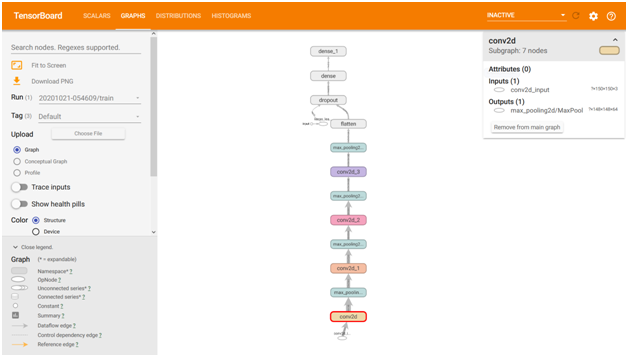
評論