Keras 是一種高級(jí)神經(jīng)網(wǎng)絡(luò)接口,可以在多個(gè)后端上運(yùn)行。其函數(shù)式 API 非常人性化且頗具靈活性,可構(gòu)建各種應(yīng)用。一經(jīng)推出,Keras 便迅速受到青睞。2017 年,Keras API 以 tf.keras 的形式實(shí)現(xiàn)與核心 TensorFlow 的集成。雖然 tf.keras 和 Keras 擁有獨(dú)立代碼庫(kù),但彼此之間緊密耦合。自 TensorFlow 1.9 起發(fā)布更新文檔和編程人員指南以來(lái),tf.keras 顯然成為以 TensorFlow 構(gòu)建神經(jīng)網(wǎng)絡(luò)時(shí)要使用的高級(jí) API。
注:更新文檔鏈接
https://www.tensorflow.org/tutorials/
編程人員指南鏈接
https://www.tensorflow.org/guide/keras
在這篇文章中,我們會(huì)介紹使用 tf.keras訓(xùn)練、導(dǎo)出及提供神經(jīng)網(wǎng)絡(luò)的整個(gè)流程。例如,我們將使用 Kaggle Planet 數(shù)據(jù)集訓(xùn)練卷積神經(jīng)網(wǎng)絡(luò),以預(yù)測(cè)亞馬遜森林衛(wèi)星圖像的標(biāo)簽。我們的目的是說(shuō)明真實(shí)用例的端到端管道。相關(guān)代碼可通過(guò)github上的可運(yùn)行筆記本獲取。請(qǐng)注意,您將需要安裝最新版本的 TensorFlow(1.11.0,每日構(gòu)建版),以根據(jù)指示完成全部操作。這只是 pip 安裝,且 requirements.txt 文件會(huì)在存儲(chǔ)區(qū)中提供?;蛘撸部梢酝ㄟ^(guò)Google Colab立即運(yùn)行內(nèi)容!
注:github 鏈接
https://github.com/sdcubber/keras-training-serving/blob/master/training-and-serving-with-tf-keras.ipynb
Google Colab 鏈接
https://colab.research.google.com/github/sdcubber/keras-training-serving/blob/master/training-and-serving-with-tf-keras.ipynb
您可在Kaggle下載數(shù)據(jù)。訓(xùn)練數(shù)據(jù)由大約 40000 張帶標(biāo)簽的亞馬遜雨林圖像組成,每張圖像均與多個(gè)標(biāo)簽關(guān)聯(lián):
注:Kaggle 鏈接
https://www.kaggle.com/c/planet-understanding-the-amazon-from-space/data
只有一個(gè) “天氣” 標(biāo)簽:晴朗、薄霧、多云或局部多云
一個(gè)或多個(gè) “地面” 標(biāo)簽:農(nóng)田、裸地、住宅、道路、水域……
天氣標(biāo)簽:多云 地面標(biāo)簽:原始森林,道路
Pandas DataFrame 包含圖像名稱列、天氣標(biāo)簽列和地面標(biāo)簽列,而系統(tǒng)會(huì)將這些數(shù)據(jù)編碼為二進(jìn)制向量。您可前往github以 .csv 文件的形式獲取相關(guān)內(nèi)容:
注:github 鏈接
https://github.com/sdcubber/keras-training-serving/blob/master/KagglePlanetMCML.csv
我們希望訓(xùn)練出的模型能夠準(zhǔn)確預(yù)測(cè)新圖像的這些標(biāo)簽。為此,我們會(huì)嘗試使用針對(duì)天氣和地面標(biāo)簽提供兩種獨(dú)立輸出的網(wǎng)絡(luò)。預(yù)測(cè)天氣標(biāo)簽是多類別分類問(wèn)題的一個(gè)例子,而地面標(biāo)簽可以建模為多標(biāo)簽分類問(wèn)題。因此,兩種輸出的損失函數(shù)有所不同。訓(xùn)練模型后,我們會(huì)使用TensorFlow Serving導(dǎo)出和提供模型,,如此一來(lái),我們就可以通過(guò) HTTP 發(fā)送請(qǐng)求以獲得圖像的預(yù)測(cè)結(jié)果。
指定模型
我們將從頭開(kāi)始構(gòu)建自己的模型1。我們會(huì)采用十分經(jīng)典的配置,包括一些卷積層、ReLU 激活函數(shù)和兩個(gè)位于頂層的密集分類器:
1import tensorflow as tf
2IM_SIZE = 128
3
4image_input = tf.keras.Input(shape=(IM_SIZE, IM_SIZE, 3), name='input_layer')
5
6# Some convolutional layers
7conv_1 = tf.keras.layers.Conv2D(32,
8kernel_size=(3, 3),
9padding='same',
10activation='relu')(image_input)
11conv_1 = tf.keras.layers.MaxPooling2D(padding='same')(conv_1)
12conv_2 = tf.keras.layers.Conv2D(32,
13kernel_size=(3, 3),
14padding='same',
15activation='relu')(conv_1)
16conv_2 = tf.keras.layers.MaxPooling2D(padding='same')(conv_2)
17
18# Flatten the output of the convolutional layers
19conv_flat = tf.keras.layers.Flatten()(conv_2)
20
21# Some dense layers with two separate outputs
22fc_1 = tf.keras.layers.Dense(128,
23activation='relu')(conv_flat)
24fc_1 = tf.keras.layers.Dropout(0.2)(fc_1)
25fc_2 = tf.keras.layers.Dense(128,
26activation='relu')(fc_1)
27fc_2 = tf.keras.layers.Dropout(0.2)(fc_2)
28
29# Output layers: separate outputs for the weather and the ground labels
30weather_output = tf.keras.layers.Dense(4,
31activation='softmax',
32name='weather')(fc_2)
33ground_output = tf.keras.layers.Dense(13,
34activation='sigmoid',
35 name='ground')(fc_2)
36
37# Wrap in a Model
38model = tf.keras.Model(inputs=image_input, outputs=[weather_output, ground_output])
我們有兩個(gè)輸出層,因此在指定模型時(shí)應(yīng)將這些層以輸出列表的形式傳遞。請(qǐng)注意,天氣和地面輸出層的激活函數(shù)并不相同。很方便的是,Model 實(shí)現(xiàn) tf.keras 時(shí)會(huì)采用簡(jiǎn)便的 summary() 方法:
編譯模型時(shí),系統(tǒng)會(huì)以字典的形式提供兩個(gè)不同的損失函數(shù),而此字典會(huì)將張量名稱映射到損失:
1model.compile(optimizer='adam',
2loss={'weather': 'categorical_crossentropy',
3'ground': 'binary_crossentropy'})
編譯模型時(shí),系統(tǒng)會(huì)以隨機(jī)權(quán)重對(duì)其進(jìn)行初始化,并允許我們選擇優(yōu)化算法來(lái)訓(xùn)練網(wǎng)絡(luò)。
[1] 正如在 Kaggle 競(jìng)賽中所證明的那樣,通過(guò)大型預(yù)訓(xùn)練網(wǎng)絡(luò)進(jìn)行遷移學(xué)習(xí)是取得成功的一大關(guān)鍵。但這里的重點(diǎn)并不是在 Kaggle 中獲勝。如需獲取有關(guān)如何實(shí)現(xiàn)絕佳性能的提示,請(qǐng)觀看介紹如何處理此數(shù)據(jù)集的精彩 fast.ai課程。
注:如何處理此數(shù)據(jù)集的精彩 fast.ai 鏈接
http://course.fast.ai/lessons/lesson3.html
模型訓(xùn)練
我們開(kāi)始訓(xùn)練模型吧!我會(huì)在我的筆記本電腦上訓(xùn)練此模型,但這臺(tái)電腦的內(nèi)存不夠,無(wú)法存儲(chǔ)整個(gè)數(shù)據(jù)集。處理圖像數(shù)據(jù)時(shí)經(jīng)常會(huì)出現(xiàn)這種情況。Keras 提供 model.fit_generator() 方法,而該方法可以使用自定義 Python 生成器從磁盤生成圖像以進(jìn)行訓(xùn)練。不過(guò),從 Keras 2.0.6 開(kāi)始,我們可以使用 Sequence 對(duì)象(而不是生成器)實(shí)現(xiàn)安全的多進(jìn)程處理,這意味著您可以顯著提升運(yùn)行速度并降低 GPU(如果您有)遇到瓶頸的風(fēng)險(xiǎn)。Keras 文檔已經(jīng)提供出色的示例代碼,我會(huì)稍微自定義一下,以實(shí)現(xiàn)下列目的:
讓其使用將圖像名稱映射到標(biāo)簽的 DataFrame
每隔一個(gè)周期打亂訓(xùn)練數(shù)據(jù)
1import ast
2import numpy as np
3import math
4import os
5import random
6from tensorflow.keras.preprocessing.image import
7img_to_array as img_to_array
8
9from tensorflow.keras.preprocessing.image import load_img as load_img
def load_image(image_path, size):
10# data augmentation logic such as random rotations can be added here
11return img_to_array(load_img(image_path, target_size=(size, size))) / 255.
12
13class KagglePlanetSequence(tf.keras.utils.Sequence):
14"""
15Custom Sequence object to train a model on out-of-memory datasets.
16"""
17
18def __init__(self, df_path, data_path, im_size, batch_size, mode='train'):
19"""
20df_path: path to a .csv file that contains columns with image names and labels
21data_path: path that contains the training images
22im_size: image size
23mode: when in training mode, data will be shuffled between epochs
24"""
25self.df = pd.read_csv(df_path)
26self.im_size = im_size
27 self.batch_size = batch_size
28self.mode = mode
29
30# Take labels and a list of image locations in memory
31self.wlabels = self.df['weather_labels'].apply(lambda x: ast.literal_eval(x)).tolist()
32self.glabels = self.df['ground_labels'].apply(lambda x: ast.literal_eval(x)).tolist()
33self.image_list = self.df['image_name'].apply(lambda x: os.path.join(data_path, x + '.jpg')).tolist()
34
35def __len__(self):
36return int(math.ceil(len(self.df) / float(self.batch_size)))
37
38def on_epoch_end(self):
39# Shuffles indexes after each epoch
40self.indexes = range(len(self.image_list))
41if self.mode == 'train':
42self.indexes = random.sample(self.indexes, k=len(self.indexes))
43
44def get_batch_labels(self, idx):
45# Fetch a batch of labels
46return [self.wlabels[idx * self.batch_size: (idx + 1) * self.batch_size],
47self.glabels[idx * self.batch_size: (idx + 1) * self.batch_size]]
48
49def get_batch_features(self, idx):
50# Fetch a batch of images
51batch_images = self.image_list[idx * self.batch_size: (1 + idx) * self.batch_size]
52return np.array([load_image(im, self.im_size) for im in batch_images])
53
54def __getitem__(self, idx):
55batch_x = self.get_batch_features(idx)
56batch_y = self.get_batch_labels(idx)
57return batch_x, batch_y
您可以使用此 Sequence 對(duì)象(而不是自定義生成器)和 fit_generator() 來(lái)訓(xùn)練模型。請(qǐng)注意,您無(wú)需提供每個(gè)周期的步驟數(shù)量,因?yàn)?__len__ 方法會(huì)實(shí)現(xiàn)生成器的相應(yīng)邏輯。
1seq = KagglePlanetSequence('./KagglePlanetMCML.csv',
2'./data/train/',
3im_size=IM_SIZE,
4batch_size=32)
此外,tf.keras 讓您可以使用所有可用的 Keras 回調(diào),以用于提升訓(xùn)練循環(huán)。這些調(diào)用非常強(qiáng)大,可提供提前停止、安排學(xué)習(xí)速率及存儲(chǔ) TensorBoard 文件等選項(xiàng)……在這里,我們將在每個(gè)周期結(jié)束后使用 ModelCheckPoint 回調(diào)來(lái)保存模型,如此一來(lái),之后我們便可在有需要時(shí)繼續(xù)訓(xùn)練。默認(rèn)情況下,系統(tǒng)會(huì)存儲(chǔ)模型架構(gòu)、訓(xùn)練配置、優(yōu)化器狀態(tài)和權(quán)重,以便通過(guò)單個(gè)文件重新創(chuàng)建整個(gè)模型。
我們開(kāi)始訓(xùn)練模型一個(gè)周期:
1callbacks = [
2tf.keras.callbacks.ModelCheckpoint('./model.h5', verbose=1)
3]
4
5model.fit_generator(generator=seq,
6verbose=1,
7epochs=1,
8use_multiprocessing=True,
9workers=4,
10callbacks=callbacks)
Epoch 1/1Epoch 00001: saving model to ./model.h51265/1265 [==============================] - 941s 744ms/step - loss: 0.8686 - weather_loss: 0.6571 - ground_loss: 0.2115
假設(shè)我們想在后期階段微調(diào)模型,只需讀取模型文件并繼續(xù)訓(xùn)練,而無(wú)需重新編譯:
1another_model = tf.keras.models.load_model('./model.h5')
2another_model.fit_generator(generator=seq, verbose=1, epochs=1)
最后,比較好的做法是,實(shí)例化 Sequencein 測(cè)試模式(即不打亂)并將其用于為整個(gè)數(shù)據(jù)集進(jìn)行預(yù)測(cè),以驗(yàn)證我們的 Sequence 是否有效傳遞所有數(shù)據(jù):
1test_seq = KagglePlanetSequence('./KagglePlanetMCML.csv',
2'./data/train/',
3im_size=IM_SIZE,
4batch_size=32, mode='test')
5predictions = model.predict_generator(generator=test_seq, verbose=1)
6len(predictions[1]) == len(df_train) # This is True!
那么,數(shù)據(jù)集 API 又是什么呢?
tf.data API 是一個(gè)功能強(qiáng)大的內(nèi)容庫(kù),讓您可以使用來(lái)自各種來(lái)源的數(shù)據(jù)并將其傳遞給 TensorFlow 模型。我們可以使用 tf.data API 而不是 Sequence 對(duì)象來(lái)訓(xùn)練 tf.keras 模型嗎?可以。首先,我們將圖像和標(biāo)簽一起序列化為 TFRecord 文件,這是在 TensorFlow 中序列化數(shù)據(jù)所推薦生成的格式:
1# Serialize images, together with labels, to TF records
2def _bytes_feature(value):
3returntf.train.Feature(bytes_list=tf.train.BytesList(value=[value]))
4
5tf_records_filename = './data/KagglePlanetTFRecord_{}'.format(IM_SIZE)
6writer = tf.python_io.TFRecordWriter(tf_records_filename)
7
8# List of image paths, np array of labels
9im_list = [os.path.join('./data/train', v + '.jpg') for v in df_train['image_name'].tolist()]
10w_labels_arr = np.array([ast.literal_eval(l) for l in df_train['weather_labels']])
11g_labels_arr = np.array([ast.literal_eval(l) for l in df_train['ground_labels']])
12
13# Loop over images and labels, wrap in TF Examples, write away to TFRecord file
14for i in range(len(df_train)):
15w_labels = w_labels_arr[i].astype(np.float32)
16g_labels = g_labels_arr[i].astype(np.float32)
17im = np.array(img_to_array(load_img(im_list[i], target_size=(IM_SIZE, IM_SIZE))) / 255.)
18
19example = tf.train.Example(features=tf.train.Features(feature={'image': _bytes_feature(im.tostring()),
20 'weather_labels': _bytes_feature(w_labels.tostring()),
21 'ground_labels': _bytes_feature(g_labels.tostring())}))
22
23writer.write(example.SerializeToString())
24
25writer.close()
將圖像和標(biāo)簽轉(zhuǎn)儲(chǔ)到 TFRecord 文件后,我們可以使用 tf.data API 設(shè)計(jì)另一個(gè)生成器。其理念是實(shí)例化我們文件中的 TFRecordDataset,并告訴它如何使用 map() 操作來(lái)解析序列化數(shù)據(jù)。
1featdef = {
2 'image': tf.FixedLenFeature(shape=[], dtype=tf.string),
3 'weather_labels': tf.FixedLenFeature(shape=[], dtype=tf.string),
4 'ground_labels': tf.FixedLenFeature(shape=[], dtype=tf.string)
5}
6
7def _parse_record(example_proto, clip=False):
8"""Parse a single record into image, weather labels, ground labels"""
9example = tf.parse_single_example(example_proto, featdef)
10im = tf.decode_raw(example['image'], tf.float32)
11im = tf.reshape(im, (IM_SIZE, IM_SIZE, 3))
12weather = tf.decode_raw(ex['weather_labels'], tf.float32)
13ground = tf.decode_raw(ex['ground_labels'], tf.float32)
14return im, weather, ground
15
16# Construct a TFRecordDataset
17ds_train = tf.data.TFRecordDataset('./data/KagglePlanetTFRecord_{}'.format(IM_SIZE)).map(_parse_record)
18ds_train = ds_train.shuffle(1000).batch(32)
Dataset 對(duì)象提供多種方法來(lái)生成迭代器對(duì)象以循環(huán)使用數(shù)據(jù)。不過(guò),從 TensorFlow 1.9 開(kāi)始,我們可以直接將 ds_train 傳遞給 model.fit() 以訓(xùn)練模型:
1model = tf.keras.Model(inputs=image_input, outputs=[weather_output, ground_output])
2
3model.compile(optimizer='adam',
4loss={'weather': 'categorical_crossentropy',
5'ground': 'binary_crossentropy'})
6
7history = model.fit(ds_train,
8 steps_per_epoch=100, # let's take just a couple of steps
9 epochs=1)
Epoch 1/1
100/100 [==============================] - 76s 755ms/step - loss: 0.5460 - weather_loss: 0.3780 - ground_loss: 0.1680
效果不錯(cuò)。這種運(yùn)作方式讓習(xí)慣使用 TFRecords 的用戶注意到 tf.keras。如果您想使用驗(yàn)證數(shù)據(jù),只需通過(guò)驗(yàn)證數(shù)據(jù)實(shí)例化另一個(gè) Dataset,再同樣傳遞給 model.fit() 即可。
提供模型
什么是提供模型?我們的目的在于:在客戶端生成輸入圖像。我們希望將此圖像包裝在某種消息中,然后將其發(fā)送到托管我們訓(xùn)練模型的遠(yuǎn)程服務(wù)器,最后從服務(wù)器接收預(yù)測(cè)作為響應(yīng)。
提供 ML 模型:客戶端發(fā)送輸入請(qǐng)求,服務(wù)器從模型中獲取預(yù)測(cè)結(jié)果并將其作為響應(yīng)發(fā)回給客戶端
首先,我們希望以服務(wù)器可以處理的格式導(dǎo)出模型。TensorFlow 提供 SavedModel 格式作為導(dǎo)出模型的通用格式。在后臺(tái),我們的 tf.keras 模型完全是根據(jù) TensorFlow 對(duì)象來(lái)指定,因此我們可以使用 TensorFlow 方法將其導(dǎo)出。
導(dǎo)出模型背后的主要理念是通過(guò)簽名定義指定推理計(jì)算。SignatureDef 完全是根據(jù)輸入和輸出張量來(lái)指定,最終會(huì)與模型權(quán)重存儲(chǔ)在一起。不過(guò),TensorFlow 提供了一個(gè)便利函數(shù) tf.saved_model.simple_save(),抽離出部分相關(guān)細(xì)節(jié),并適用于大多數(shù)用例:
1import tensorflow as tf
2
3# The export path contains the name and the version of the model
4tf.keras.backend.set_learning_phase(0) # Ignore dropout at inference
5model = tf.keras.models.load_model('./model.h5')
6export_path = './PlanetModel/1'
7
8# Fetch the Keras session and save the model
9# The signature definition is defined by the input and output tensors
10 # And stored with the default serving key
11with tf.keras.backend.get_session() as sess:
12tf.saved_model.simple_save(
13sess,
14export_path,
15inputs={'input_image': model.input},
16outputs={t.name:t for t in model.outputs})
INFO:tensorflow:No assets to save.INFO:tensorflow:No assets to write.INFO:tensorflow:SavedModel written to: ./PlanetModel/1/saved_model.pb
請(qǐng)注意,我已在導(dǎo)出路徑中指定版本號(hào)。原因在于,TensorFlow Serving 會(huì)根據(jù)存儲(chǔ)目錄的名稱推斷出模型版本。如果我們想出更好的模型,便可將其存儲(chǔ)在 PlanetModel/2 下,而 TF Serving 會(huì)自動(dòng)更新以托管新模型。模型圖會(huì)存儲(chǔ)在版本子目錄中,而變量則存儲(chǔ)在另一個(gè)子目錄中:
$ tree
.└── 1 ├── saved_model.pb └── variables ├── variables.data-00000-of-00001 └── variables.index
在設(shè)置真實(shí)服務(wù)器之前,我想強(qiáng)調(diào)一下 TensorFlow 的 SavedModel 命令行工具,其有助于快速檢查我們模型的輸入和輸出規(guī)格:
$ saved_model_cli show --dir ./ --allThe given SavedModel SignatureDef contains the following input(s): inputs['input_image'] tensor_info: dtype: DT_FLOAT shape: (-1, 128, 128, 3) name: input_layer_2:0The given SavedModel SignatureDef contains the following output(s): outputs['ground_2/Sigmoid:0'] tensor_info: dtype: DT_FLOAT shape: (-1, 13) name: ground_2/Sigmoid:0 outputs['weather_2/Softmax:0'] tensor_info: dtype: DT_FLOAT shape: (-1, 4) name: weather_2/Softmax:0Method name is: tensorflow/serving/predict
我們甚至可以訪問(wèn) CLI 中的 Numpy(即 np),以向模型發(fā)送一些隨機(jī)輸入,進(jìn)而驗(yàn)證模型是否可正常運(yùn)作:
$ saved_model_cli run --dir ./ --tag_set serve --signature_def serving_default --input_exp 'input_image=np.random.rand(1,128,128,3)'
Result for output key ground_2/Sigmoid:0:[[6.5955728e-01 9.8123280e-03 1.4992488e-02 1.9942504e-06 3.5892407e-07 3.2538961e-04 2.4094069e-02 6.0808718e-01 9.8486900e-01 7.9137814e-01 1.4336356e-05 1.6872218e-05 3.8697788e-01]]Result for output key weather_2/Softmax:0:[[7.1896911e-01 2.9373894e-04 2.5214682e-05 2.8071195e-01]]
看來(lái)可以正常運(yùn)作!
使用 TensorFlow Serving 托管模型服務(wù)器
我們會(huì)使用TensorFlow Serving內(nèi)容庫(kù)來(lái)托管模型:
TensorFlow Serving 是一種靈活的高性能服務(wù)系統(tǒng),適用于機(jī)器學(xué)習(xí)模型,并專為生產(chǎn)環(huán)境而設(shè)計(jì)。
Servable是 TensorFlow Serving 的核心抽象概念,并將代表模型。除此之外,TF Serving還提供處理實(shí)際服務(wù)、加載新版本和卸載舊版本的來(lái)源、加載器和管理器。
在本教程中,我們將在本地設(shè)置服務(wù)器。在生產(chǎn)環(huán)境中,您可以在某些微服務(wù)架構(gòu)(例如,在 Kubernetes 集群的 pod 上)中以完全相同的方式設(shè)置服務(wù)器。
只需一個(gè)命令,您便可以在頂層模型目錄中托管模型。我截?cái)嗔艘恍┹敵霾⑼怀鲲@示 TF Serving 后端的一些組件,如下所示:
$ tensorflow_model_server --model_base_path=$(pwd) --rest_api_port=9000 --model_name=PlanetModelI tensorflow_serving/core/basic_manager] Successfully reserved resources to load servable {name: PlanetModel version: 1}I tensorflow_serving/core/loader_harness.cc] Loading servable version {name: PlanetModel version: 1}I external/org_tensorflow/tensorflow/cc/saved_model/loader.cc] Loading SavedModel with tags: { serve };I external/org_tensorflow/tensorflow/cc/saved_model/loader.cc] SavedModel load for tags { serve }; Status: success. Took 1048518 microseconds.I tensorflow_serving/core/loader_harness.cc] Successfully loaded servable version {name: PlanetModel version: 1}I tensorflow_serving/model_servers/main.cc] Exporting HTTP/REST API at:localhost:9000 ...
在服務(wù)器啟動(dòng)并運(yùn)行時(shí),我們可以向其發(fā)出請(qǐng)求。從 TensorFlow Serving 1.8 開(kāi)始,我們可以通過(guò) gRPC 或 HTTP 調(diào)用受托管模型。這兩種情況的理念相同:我們希望填充有效負(fù)載消息并將其發(fā)送給服務(wù)器,然后服務(wù)器應(yīng)返回包含預(yù)測(cè)結(jié)果的消息。對(duì)于 gRPC,有一些 Python 綁定可用于填充 protobuf 文件格式的消息。如要發(fā)送 HTTP 請(qǐng)求,只需使用 Python requests 模塊在有效負(fù)載 json 中包裝我們的輸出:
1import requests
2import json
3
4image = img_to_array(load_img('./data/train/train_10001.jpg', target_size=(128,128))) / 255.
5payload = {
6"instances": [{'input_image': image.tolist()}]
7}
8r = requests.post('http://localhost:9000/v1/models/PlanetModel:predict', json=payload)
9json.loads(r.content)
請(qǐng)求網(wǎng)址是根據(jù) TF Serving 文檔中所述的一些規(guī)則而組成的。發(fā)送請(qǐng)求后,服務(wù)器會(huì)立即返回天氣和地面標(biāo)簽的輸出列表:
{u'predictions': [ {u'ground_2/Sigmoid:0': [ 0.153237, 0.000527727, 0.00555856, 0.00542973, 0.00105254, 0.000256282, 0.103614, 0.0325185, 0.998204, 0.072204, 0.00745501, 0.00326175, 0.0942268], u'weather_2/Softmax:0': [ 0.963947, 0.000207846, 0.00113924, 0.0347063] }]}
預(yù)測(cè):原始森林天氣晴朗,但缺少農(nóng)田和道路的天氣狀況。請(qǐng)返回訓(xùn)練
總結(jié)
tf.keras 讓 TensorFlow 用戶可以充分利用 Keras 的全部功能和靈活性。tf.keras 使用起來(lái)很有趣,其與核心 TensorFlow 的集成絕對(duì)使我們向更廣泛的受眾提供深度學(xué)習(xí)這一目標(biāo)向前邁進(jìn)一大步。事實(shí)上,它們像任何其他 TF 模型一樣,能夠以 SavedModel 格式導(dǎo)出并使用 TensorFlow Serving 提供模型,這使在生產(chǎn)環(huán)境中使用 tf.keras 變得簡(jiǎn)單直觀。
-
神經(jīng)網(wǎng)絡(luò)
+關(guān)注
關(guān)注
42文章
4772瀏覽量
100845 -
圖像
+關(guān)注
關(guān)注
2文章
1085瀏覽量
40490 -
tensorflow
+關(guān)注
關(guān)注
13文章
329瀏覽量
60537
原文標(biāo)題:使用 tf.keras 訓(xùn)練和提供 ML 模型
文章出處:【微信號(hào):tensorflowers,微信公眾號(hào):Tensorflowers】歡迎添加關(guān)注!文章轉(zhuǎn)載請(qǐng)注明出處。
發(fā)布評(píng)論請(qǐng)先 登錄
相關(guān)推薦
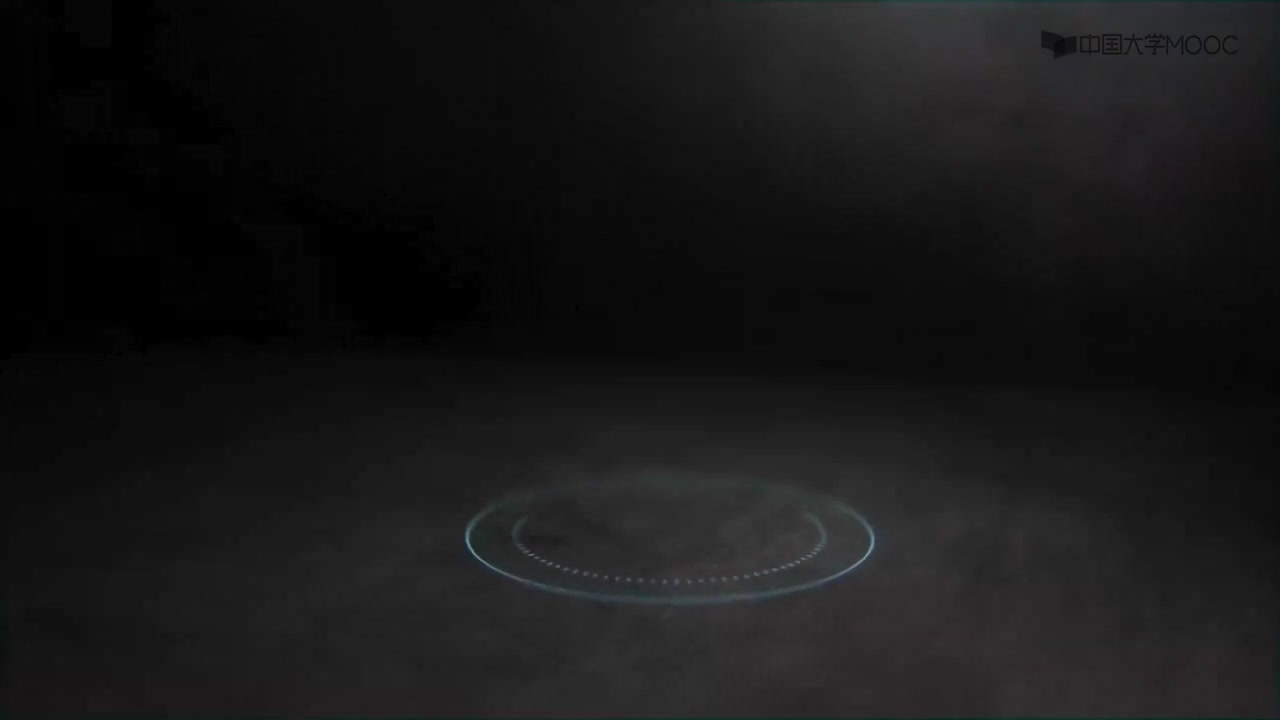
3 keras和tf.keras(1)#神經(jīng)網(wǎng)絡(luò)
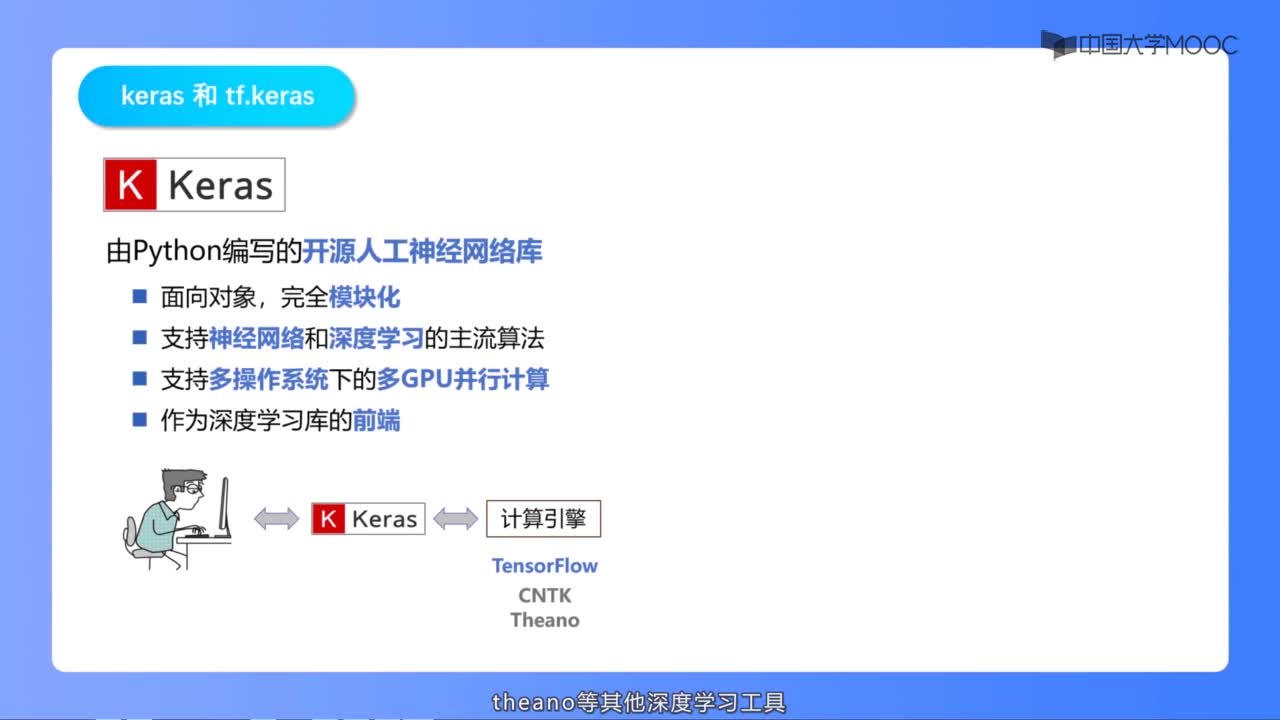
評(píng)論