1. Take-Away
筆者使用 PyTorch 編寫了不同加速庫在 ImageNet 上的使用示例(單機(jī)多卡)。需要的同學(xué)可以當(dāng)作 quickstart 將所需要的部分 copy 到自己的項(xiàng)目中(Github 請點(diǎn)擊下面鏈接):
nn.DataParallel[1] 簡單方便的 nn.DataParallel
torch.distributed[2] 使用 torch.distributed 加速并行訓(xùn)練
torch.multiprocessing[3] 使用 torch.multiprocessing 取代啟動器
apex[4] 使用 apex 再加速
horovod[5] horovod 的優(yōu)雅實(shí)現(xiàn)
注:分布式 evaluation[6]
這里,筆者記錄了使用 4 塊 Tesla V100-PICE 在 ImageNet 進(jìn)行了運(yùn)行時間的測試。
測試結(jié)果發(fā)現(xiàn) Apex 的加速效果最好,但與 Horovod/Distributed 差別不大,平時可以直接使用內(nèi)置的 Distributed。Dataparallel 較慢,不推薦使用。
(后續(xù)會補(bǔ)上 V100/K80 上的測試結(jié)果,穿插了一些試驗(yàn)所以中斷了。)
簡要記錄一下不同庫的分布式訓(xùn)練方式,當(dāng)作代碼的 README(我真是個小機(jī)靈鬼)~
2. 簡單方便的 nn.DataParallel
DataParallel 可以幫助我們(使用單進(jìn)程控)將模型和數(shù)據(jù)加載到多個 GPU 中,控制數(shù)據(jù)在 GPU 之間的流動,協(xié)同不同 GPU 上的模型進(jìn)行并行訓(xùn)練(細(xì)粒度的方法有 scatter,gather 等等)。
DataParallel 使用起來非常方便,我們只需要用 DataParallel 包裝模型,再設(shè)置一些參數(shù)即可。
需要定義的參數(shù)包括:
參與訓(xùn)練的 GPU 有哪些,device_ids=gpus;
用于匯總梯度的 GPU 是哪個,output_device=gpus[0] 。
DataParallel 會自動幫我們將數(shù)據(jù)切分 load 到相應(yīng) GPU,將模型復(fù)制到相應(yīng) GPU,進(jìn)行正向傳播計(jì)算梯度并匯總:
model = nn.DataParallel(model.cuda(), device_ids=gpus, output_device=gpus[0])
值得注意的是,模型和數(shù)據(jù)都需要先 load 進(jìn) GPU 中,DataParallel 的 module 才能對其進(jìn)行處理,否則會報錯:
# 這里要 model.cuda() model = nn.DataParallel(model.cuda(), device_ids=gpus, output_device=gpus[0]) for epoch in range(100): for batch_idx, (data, target) in enumerate(train_loader): # 這里要 images/target.cuda() images = images.cuda(non_blocking=True) target = target.cuda(non_blocking=True) ... output = model(images) loss = criterion(output, target) ... optimizer.zero_grad() loss.backward() optimizer.step()
匯總一下,DataParallel 并行訓(xùn)練部分主要與如下代碼段有關(guān):
# main.py import torch import torch.distributed as dist gpus = [0, 1, 2, 3] torch.cuda.set_device('cuda:{}'.format(gpus[0])) train_dataset = ... train_loader = torch.utils.data.DataLoader(train_dataset, batch_size=...) model = ... model = nn.DataParallel(model.to(device), device_ids=gpus, output_device=gpus[0]) optimizer = optim.SGD(model.parameters()) for epoch in range(100): for batch_idx, (data, target) in enumerate(train_loader): images = images.cuda(non_blocking=True) target = target.cuda(non_blocking=True) ... output = model(images) loss = criterion(output, target) ... optimizer.zero_grad() loss.backward() optimizer.step()
在使用時,使用 python 執(zhí)行即可:
python main.py
在 ImageNet 上的完整訓(xùn)練代碼,請點(diǎn)擊Github[7]。
3. 使用 torch.distributed 加速并行訓(xùn)練
在 pytorch 1.0 之后,官方終于對分布式的常用方法進(jìn)行了封裝,支持 all-reduce,broadcast,send 和 receive 等等。通過 MPI 實(shí)現(xiàn) CPU 通信,通過 NCCL 實(shí)現(xiàn) GPU 通信。官方也曾經(jīng)提到用 DistributedDataParallel 解決 DataParallel 速度慢,GPU 負(fù)載不均衡的問題,目前已經(jīng)很成熟了~
與 DataParallel 的單進(jìn)程控制多 GPU 不同,在 distributed 的幫助下,我們只需要編寫一份代碼,torch 就會自動將其分配給 n 個進(jìn)程,分別在 n 個 GPU 上運(yùn)行。
在 API 層面,pytorch 為我們提供了 torch.distributed.launch 啟動器,用于在命令行分布式地執(zhí)行 python 文件。在執(zhí)行過程中,啟動器會將當(dāng)前進(jìn)程的(其實(shí)就是 GPU的)index 通過參數(shù)傳遞給 python,我們可以這樣獲得當(dāng)前進(jìn)程的 index:
parser = argparse.ArgumentParser() parser.add_argument('--local_rank', default=-1, type=int, help='node rank for distributed training') args = parser.parse_args() print(args.local_rank)
接著,使用 init_process_group 設(shè)置GPU 之間通信使用的后端和端口:
dist.init_process_group(backend='nccl')
之后,使用 DistributedSampler 對數(shù)據(jù)集進(jìn)行劃分。如此前我們介紹的那樣,它能幫助我們將每個 batch 劃分成幾個 partition,在當(dāng)前進(jìn)程中只需要獲取和 rank 對應(yīng)的那個 partition 進(jìn)行訓(xùn)練:
train_sampler = torch.utils.data.distributed.DistributedSampler(train_dataset) train_loader = torch.utils.data.DataLoader(train_dataset, batch_size=..., sampler=train_sampler)
然后,使用 DistributedDataParallel 包裝模型,它能幫助我們?yōu)椴煌?GPU 上求得的梯度進(jìn)行 all reduce(即匯總不同 GPU 計(jì)算所得的梯度,并同步計(jì)算結(jié)果)。all reduce 后不同 GPU 中模型的梯度均為 all reduce 之前各 GPU 梯度的均值:
model = torch.nn.parallel.DistributedDataParallel(model, device_ids=[args.local_rank])
最后,把數(shù)據(jù)和模型加載到當(dāng)前進(jìn)程使用的 GPU 中,正常進(jìn)行正反向傳播:
torch.cuda.set_device(args.local_rank) model.cuda() for epoch in range(100): for batch_idx, (data, target) in enumerate(train_loader): images = images.cuda(non_blocking=True) target = target.cuda(non_blocking=True) ... output = model(images) loss = criterion(output, target) ... optimizer.zero_grad() loss.backward() optimizer.step()
匯總一下,torch.distributed 并行訓(xùn)練部分主要與如下代碼段有關(guān):
# main.py import torch import argparse import torch.distributed as dist parser = argparse.ArgumentParser() parser.add_argument('--local_rank', default=-1, type=int, help='node rank for distributed training') args = parser.parse_args() dist.init_process_group(backend='nccl') torch.cuda.set_device(args.local_rank) train_dataset = ... train_sampler = torch.utils.data.distributed.DistributedSampler(train_dataset) train_loader = torch.utils.data.DataLoader(train_dataset, batch_size=..., sampler=train_sampler) model = ... model = torch.nn.parallel.DistributedDataParallel(model, device_ids=[args.local_rank]) optimizer = optim.SGD(model.parameters()) for epoch in range(100): for batch_idx, (data, target) in enumerate(train_loader): images = images.cuda(non_blocking=True) target = target.cuda(non_blocking=True) ... output = model(images) loss = criterion(output, target) ... optimizer.zero_grad() loss.backward() optimizer.step()
在使用時,調(diào)用 torch.distributed.launch 啟動器啟動:
CUDA_VISIBLE_DEVICES=0,1,2,3 python -m torch.distributed.launch --nproc_per_node=4 main.py
在 ImageNet 上的完整訓(xùn)練代碼,請點(diǎn)擊Github[8]。
4. 使用 torch.multiprocessing 取代啟動器
有的同學(xué)可能比較熟悉 torch.multiprocessing,也可以手動使用 torch.multiprocessing 進(jìn)行多進(jìn)程控制。繞開 torch.distributed.launch 自動控制開啟和退出進(jìn)程的一些小毛病~
使用時,只需要調(diào)用 torch.multiprocessing.spawn,torch.multiprocessing 就會幫助我們自動創(chuàng)建進(jìn)程。
如下面的代碼所示,spawn 開啟了 nprocs=4 個進(jìn)程,每個進(jìn)程執(zhí)行 main_worker 并向其中傳入 local_rank(當(dāng)前進(jìn)程 index)和 args(即 4 和 myargs)作為參數(shù):
import torch.multiprocessing as mp mp.spawn(main_worker, nprocs=4, args=(4, myargs))
這里,我們直接將原本需要 torch.distributed.launch 管理的執(zhí)行內(nèi)容,封裝進(jìn) main_worker 函數(shù)中,其中 proc 對應(yīng) local_rank(當(dāng)前進(jìn)程 index),進(jìn)程數(shù) nprocs 對應(yīng) 4, args 對應(yīng) myargs:
def main_worker(proc, nprocs, args): dist.init_process_group(backend='nccl', init_method='tcp://127.0.0.1:23456', world_size=4, rank=gpu) torch.cuda.set_device(args.local_rank) train_dataset = ... train_sampler = torch.utils.data.distributed.DistributedSampler(train_dataset) train_loader = torch.utils.data.DataLoader(train_dataset, batch_size=..., sampler=train_sampler) model = ... model = torch.nn.parallel.DistributedDataParallel(model, device_ids=[args.local_rank]) optimizer = optim.SGD(model.parameters()) for epoch in range(100): for batch_idx, (data, target) in enumerate(train_loader): images = images.cuda(non_blocking=True) target = target.cuda(non_blocking=True) ... output = model(images) loss = criterion(output, target) ... optimizer.zero_grad() loss.backward() optimizer.step()
在上面的代碼中值得注意的是,由于沒有 torch.distributed.launch 讀取的默認(rèn)環(huán)境變量作為配置,我們需要手動為 init_process_group 指定參數(shù):
dist.init_process_group(backend='nccl', init_method='tcp://127.0.0.1:23456', world_size=4, rank=gpu)
匯總一下,添加 multiprocessing 后并行訓(xùn)練部分主要與如下代碼段有關(guān):
# main.py import torch import torch.distributed as dist import torch.multiprocessing as mp mp.spawn(main_worker, nprocs=4, args=(4, myargs)) def main_worker(proc, nprocs, args): dist.init_process_group(backend='nccl', init_method='tcp://127.0.0.1:23456', world_size=4, rank=gpu) torch.cuda.set_device(args.local_rank) train_dataset = ... train_sampler = torch.utils.data.distributed.DistributedSampler(train_dataset) train_loader = torch.utils.data.DataLoader(train_dataset, batch_size=..., sampler=train_sampler) model = ... model = torch.nn.parallel.DistributedDataParallel(model, device_ids=[args.local_rank]) optimizer = optim.SGD(model.parameters()) for epoch in range(100): for batch_idx, (data, target) in enumerate(train_loader): images = images.cuda(non_blocking=True) target = target.cuda(non_blocking=True) ... output = model(images) loss = criterion(output, target) ... optimizer.zero_grad() loss.backward() optimizer.step()
在使用時,直接使用 python 運(yùn)行就可以了:
python main.py
在 ImageNet 上的完整訓(xùn)練代碼,請點(diǎn)擊Github[9]。
5.使用 Apex 再加速
Apex 是 NVIDIA 開源的用于混合精度訓(xùn)練和分布式訓(xùn)練庫。Apex 對混合精度訓(xùn)練的過程進(jìn)行了封裝,改兩三行配置就可以進(jìn)行混合精度的訓(xùn)練,從而大幅度降低顯存占用,節(jié)約運(yùn)算時間。此外,Apex 也提供了對分布式訓(xùn)練的封裝,針對 NVIDIA 的 NCCL 通信庫進(jìn)行了優(yōu)化。
在混合精度訓(xùn)練上,Apex 的封裝十分優(yōu)雅。直接使用 amp.initialize 包裝模型和優(yōu)化器,apex 就會自動幫助我們管理模型參數(shù)和優(yōu)化器的精度了,根據(jù)精度需求不同可以傳入其他配置參數(shù)。
from apex import amp model, optimizer = amp.initialize(model, optimizer)
在分布式訓(xùn)練的封裝上,Apex 在膠水層的改動并不大,主要是優(yōu)化了 NCCL 的通信。因此,大部分代碼仍與 torch.distributed 保持一致。使用的時候只需要將 torch.nn.parallel.DistributedDataParallel 替換為 apex.parallel.DistributedDataParallel 用于包裝模型。在 API 層面,相對于 torch.distributed ,它可以自動管理一些參數(shù)(可以少傳一點(diǎn)):
from apex.parallel import DistributedDataParallel model = DistributedDataParallel(model) # # torch.distributed # model = torch.nn.parallel.DistributedDataParallel(model, device_ids=[args.local_rank]) # model = torch.nn.parallel.DistributedDataParallel(model, device_ids=[args.local_rank], output_device=args.local_rank)
在正向傳播計(jì)算 loss 時,Apex 需要使用 amp.scale_loss 包裝,用于根據(jù) loss 值自動對精度進(jìn)行縮放:
with amp.scale_loss(loss, optimizer) as scaled_loss: scaled_loss.backward()
匯總一下,Apex 的并行訓(xùn)練部分主要與如下代碼段有關(guān):
# main.py import torch import argparse import torch.distributed as dist from apex.parallel import DistributedDataParallel parser = argparse.ArgumentParser() parser.add_argument('--local_rank', default=-1, type=int, help='node rank for distributed training') args = parser.parse_args() dist.init_process_group(backend='nccl') torch.cuda.set_device(args.local_rank) train_dataset = ... train_sampler = torch.utils.data.distributed.DistributedSampler(train_dataset) train_loader = torch.utils.data.DataLoader(train_dataset, batch_size=..., sampler=train_sampler) model = ... model, optimizer = amp.initialize(model, optimizer) model = DistributedDataParallel(model, device_ids=[args.local_rank]) optimizer = optim.SGD(model.parameters()) for epoch in range(100): for batch_idx, (data, target) in enumerate(train_loader): images = images.cuda(non_blocking=True) target = target.cuda(non_blocking=True) ... output = model(images) loss = criterion(output, target) optimizer.zero_grad() with amp.scale_loss(loss, optimizer) as scaled_loss: scaled_loss.backward() optimizer.step()
在使用時,調(diào)用 torch.distributed.launch 啟動器啟動:
UDA_VISIBLE_DEVICES=0,1,2,3 python -m torch.distributed.launch --nproc_per_node=4 main.py
在 ImageNet 上的完整訓(xùn)練代碼,請點(diǎn)擊Github[10]。
6.Horovod 的優(yōu)雅實(shí)現(xiàn)
Horovod 是 Uber 開源的深度學(xué)習(xí)工具,它的發(fā)展吸取了 Facebook "Training ImageNet In 1 Hour" 與百度 "Ring Allreduce" 的優(yōu)點(diǎn),可以無痛與 PyTorch/Tensorflow 等深度學(xué)習(xí)框架結(jié)合,實(shí)現(xiàn)并行訓(xùn)練。
在 API 層面,Horovod 和 torch.distributed 十分相似。在 mpirun 的基礎(chǔ)上,Horovod 提供了自己封裝的 horovodrun 作為啟動器。
與 torch.distributed.launch 相似,我們只需要編寫一份代碼,horovodrun 啟動器就會自動將其分配給 n 個進(jìn)程,分別在 n 個 GPU 上運(yùn)行。在執(zhí)行過程中,啟動器會將當(dāng)前進(jìn)程的(其實(shí)就是 GPU的)index 注入 hvd,我們可以這樣獲得當(dāng)前進(jìn)程的 index:
import horovod.torch as hvd hvd.local_rank()
與 init_process_group 相似,Horovod 使用 init 設(shè)置GPU 之間通信使用的后端和端口:
hvd.init()
接著,使用 DistributedSampler 對數(shù)據(jù)集進(jìn)行劃分。如此前我們介紹的那樣,它能幫助我們將每個 batch 劃分成幾個 partition,在當(dāng)前進(jìn)程中只需要獲取和 rank 對應(yīng)的那個 partition 進(jìn)行訓(xùn)練:
train_sampler = torch.utils.data.distributed.DistributedSampler(train_dataset) train_loader = torch.utils.data.DataLoader(train_dataset, batch_size=..., sampler=train_sampler)
之后,使用 broadcast_parameters 包裝模型參數(shù),將模型參數(shù)從編號為 root_rank 的 GPU 復(fù)制到所有其他 GPU 中:
hvd.broadcast_parameters(model.state_dict(), root_rank=0)
然后,使用 DistributedOptimizer 包裝優(yōu)化器。它能幫助我們?yōu)椴煌?GPU 上求得的梯度進(jìn)行 all reduce(即匯總不同 GPU 計(jì)算所得的梯度,并同步計(jì)算結(jié)果)。all reduce 后不同 GPU 中模型的梯度均為 all reduce 之前各 GPU 梯度的均值:
hvd.DistributedOptimizer(optimizer, named_parameters=model.named_parameters(), compression=hvd.Compression.fp16)
最后,把數(shù)據(jù)加載到當(dāng)前 GPU 中。在編寫代碼時,我們只需要關(guān)注正常進(jìn)行正向傳播和反向傳播:
torch.cuda.set_device(args.local_rank) for epoch in range(100): for batch_idx, (data, target) in enumerate(train_loader): images = images.cuda(non_blocking=True) target = target.cuda(non_blocking=True) ... output = model(images) loss = criterion(output, target) ... optimizer.zero_grad() loss.backward() optimizer.step()
匯總一下,Horovod 的并行訓(xùn)練部分主要與如下代碼段有關(guān):
# main.py import torch import horovod.torch as hvd hvd.init() torch.cuda.set_device(hvd.local_rank()) train_dataset = ... train_sampler = torch.utils.data.distributed.DistributedSampler( train_dataset, num_replicas=hvd.size(), rank=hvd.rank()) train_loader = torch.utils.data.DataLoader(train_dataset, batch_size=..., sampler=train_sampler) model = ... model.cuda() optimizer = optim.SGD(model.parameters()) optimizer = hvd.DistributedOptimizer(optimizer, named_parameters=model.named_parameters()) hvd.broadcast_parameters(model.state_dict(), root_rank=0) for epoch in range(100): for batch_idx, (data, target) in enumerate(train_loader): images = images.cuda(non_blocking=True) target = target.cuda(non_blocking=True) ... output = model(images) loss = criterion(output, target) ... optimizer.zero_grad() loss.backward() optimizer.step()
在使用時,調(diào)用 horovodrun 啟動器啟動:
CUDA_VISIBLE_DEVICES=0,1,2,3 horovodrun -np 4 -H localhost:4 --verbose python main.py
在 ImageNet 上的完整訓(xùn)練代碼,請點(diǎn)擊Github[11]。
7.分布式 evaluation
all_reduce, barrier 等 API 是 distributed 中更為基礎(chǔ)和底層的 API。這些 API 可以幫助我們控制進(jìn)程之間的交互,控制 GPU 數(shù)據(jù)的傳輸。在自定義 GPU 協(xié)作邏輯,匯總 GPU 間少量的統(tǒng)計(jì)信息時,大有用處。熟練掌握這些 API 也可以幫助我們自己設(shè)計(jì)、優(yōu)化分布式訓(xùn)練、測試流程。
最近,不少同學(xué)私信了我這樣的問題,
訓(xùn)練樣本被切分成了若干個部分,被若干個進(jìn)程分別控制運(yùn)行在若干個 GPU 上,如何在進(jìn)程間進(jìn)行通信匯總這些(GPU 上的)信息?
使用一張卡進(jìn)行推理、測試太慢了,如何使用 Distributed 進(jìn)行分布式地推理和測試,并將結(jié)果匯總在一起?
......
要解決這些問題,我們需要一個更為基礎(chǔ)的 API,匯總記錄不同 GPU 上生成的準(zhǔn)確率、損失函數(shù)等指標(biāo)信息。這個 API 就是 torch.distributed.all_reduce。
圖2:all_reduce 示意圖
如上圖所示,它的工作過程包含以下三步:
在調(diào)用 all_reduce(tensor, op=...)后,當(dāng)前進(jìn)程會向其他進(jìn)程發(fā)送 tensor(例如 rank 0 會發(fā)送 rank 0 的 tensor 到 rank 1、2、3)
同時,當(dāng)前進(jìn)程接受其他進(jìn)程發(fā)來的 tensor(例如 rank 0 會接收 rank 1 的 tensor、rank 2 的 tensor、rank 3 的 tensor)。
在全部接收完成后,當(dāng)前進(jìn)程(例如rank 0)會對當(dāng)前進(jìn)程的和接收到的 tensor (例如 rank 0 的 tensor、rank 1 的 tensor、rank 2 的 tensor、rank 3 的 tensor)進(jìn)行 op (例如求和)操作。
使用 torch.distributed.all_reduce(loss, op=torch.distributed.reduce_op.SUM),我們就能夠?qū)Σ煌瑪?shù)據(jù)切片(不同 GPU 上的訓(xùn)練數(shù)據(jù))的損失函數(shù)進(jìn)行求和了。接著,我們只要再將其除以進(jìn)程(GPU)數(shù)量 world_size就可以得到損失函數(shù)的平均值。正確率也能夠通過同樣方法進(jìn)行計(jì)算:
# 原始代碼 output = model(images) loss = criterion(output, target) acc1, acc5 = accuracy(output, target, topk=(1, 5)) losses.update(loss.item(), images.size(0)) top1.update(acc1.item(), images.size(0)) top5.update(acc5.item(), images.size(0)) # 修改后,同步各 GPU 中數(shù)據(jù)切片的統(tǒng)計(jì)信息,用于分布式的 evaluation def reduce_tensor(tensor): rt = tensor.clone() dist.all_reduce(rt, op=dist.reduce_op.SUM) rt /= args.world_size return rt output = model(images) loss = criterion(output, target) acc1, acc5 = accuracy(output, target, topk=(1, 5)) torch.distributed.barrier() reduced_loss = reduce_tensor(loss.data) reduced_acc1 = reduce_tensor(acc1) reduced_acc5 = reduce_tensor(acc5) losses.update(loss.item(), images.size(0)) top1.update(acc1.item(), images.size(0)) top5.update(acc5.item(), images.size(0))
值得注意的是,為了同步各進(jìn)程的計(jì)算進(jìn)度,我們在 reduce 之前插入了一個同步 API torch.distributed.barrier()。在所有進(jìn)程運(yùn)行到這一步之前,先完成此前代碼的進(jìn)程會等待其他進(jìn)程。這使得我們能夠得到準(zhǔn)確、有序的輸出。在 Horovod 中,我們無法使用 torch.distributed.barrier(),取而代之的是,我們可以在 allreduce 過程中指明:
def reduce_mean(tensor, world_size): rt = tensor.clone() hvd.allreduce(rt, name='barrier') rt /= world_size return rt output = model(images) loss = criterion(output, target) acc1, acc5 = accuracy(output, target, topk=(1, 5)) reduced_loss = reduce_tensor(loss.data) reduced_acc1 = reduce_tensor(acc1) reduced_acc5 = reduce_tensor(acc5) losses.update(loss.item(), images.size(0)) top1.update(acc1.item(), images.size(0)) top5.update(acc5.item(), images.size(0))
在 ImageNet 上的完整訓(xùn)練代碼,請點(diǎn)擊Github[12]。
8.尾注:
本文中使用的 V100-PICE (前 4 個 GPU)的配置:
圖 3:配置詳情
本文中使用的 V100 (前 4 個 GPU)的配置:
圖 4:配置詳情
本文中使用的 K80 (前 4 個 GPU)的配置:
圖 5:配置詳情
筆者本身是 CV 研究生,今天摸魚的時候一時興起研究了一下,后面再慢慢完善~
-
數(shù)據(jù)
+關(guān)注
關(guān)注
8文章
7077瀏覽量
89161 -
gpu
+關(guān)注
關(guān)注
28文章
4749瀏覽量
129034 -
模型
+關(guān)注
關(guān)注
1文章
3255瀏覽量
48907
原文標(biāo)題:8.尾注:
文章出處:【微信號:zenRRan,微信公眾號:深度學(xué)習(xí)自然語言處理】歡迎添加關(guān)注!文章轉(zhuǎn)載請注明出處。
發(fā)布評論請先 登錄
相關(guān)推薦
分布式軟件系統(tǒng)
分布式數(shù)據(jù)庫有什么優(yōu)缺點(diǎn)?
HarmonyOS應(yīng)用開發(fā)-分布式設(shè)計(jì)
HarmonyOS分布式數(shù)據(jù)庫,為啥這么牛?
各種分布式電源的電氣特性
【木棉花】分布式數(shù)據(jù)庫
分布式數(shù)據(jù)庫,什么是分布式數(shù)據(jù)庫
Redis 分布式鎖的正確實(shí)現(xiàn)方式
為什么我們需要分布式數(shù)據(jù)庫
數(shù)據(jù)庫如何走向分布式
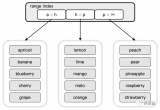
探究超大Transformer語言模型的分布式訓(xùn)練框架
**分布式數(shù)據(jù)庫|數(shù)據(jù)庫數(shù)據(jù)類型**
基于PyTorch的模型并行分布式訓(xùn)練Megatron解析
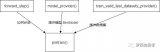
分布式鎖的三種實(shí)現(xiàn)方式
分布式通信的原理和實(shí)現(xiàn)高效分布式通信背后的技術(shù)NVLink的演進(jìn)
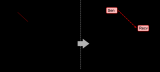
評論