引入
大家在使用谷歌或者百度搜索時,輸入搜索內(nèi)容時,谷歌總是能提供非常好的拼寫檢查,比如你輸入speling,谷歌會馬上返回spelling。
下面是用21行python代碼實現(xiàn)的一個簡易但是具備完整功能的拼寫檢查器。
代碼
import re, collections
def words(text): return re.findall('[a-z]+', text.lower())
def train(features):
model = collections.defaultdict(lambda: 1)
for f in features:
model[f] += 1
return model
NWORDS = train(words(file('big.txt').read()))
alphabet = 'abcdefghijklmnopqrstuvwxyz'
def edits1(word):
splits = [(word[:i], word[i:]) for i in range(len(word) + 1)]
deletes = [a + b[1:] for a, b in splits if b]
transposes = [a + b[1] + b[0] + b[2:] for a, b in splits if len(b)>1]
replaces = [a + c + b[1:] for a, b in splits for c in alphabet if b]
inserts = [a + c + b for a, b in splits for c in alphabet]
return set(deletes + transposes + replaces + inserts)
def known_edits2(word):
return set(e2 for e1 in edits1(word) for e2 in edits1(e1) if e2 in NWORDS)
def known(words): return set(w for w in words if w in NWORDS)
def correct(word):
candidates = known([word]) or known(edits1(word)) or known_edits2(word) or [word]
return max(candidates, key=NWORDS.get)
correct函數(shù)是程序的入口,傳進去錯誤拼寫的單詞會返回正確。如:
>>> correct("cpoy")
'copy'
>>> correct("engilsh")
'english'
>>> correct("sruprise")
'surprise'
除了這段代碼外,作為機器學(xué)習(xí)的一部分,肯定還應(yīng)該有大量的樣本數(shù)據(jù),準(zhǔn)備了big.txt作為我們的樣本數(shù)據(jù)。
背后原理
上面的代碼是基于貝葉斯來實現(xiàn)的,事實上谷歌百度實現(xiàn)的拼寫檢查也是通過貝葉斯實現(xiàn),不過肯定比這個復(fù)雜多了。
首先簡單介紹一下背后的原理,如果讀者之前了解過了,可以跳過這段。
給一個詞,我們試圖選取一個最可能的正確的的拼寫建議(建議也可能就是輸入的單詞)。有時也不清楚(比如lates應(yīng)該被更正為late或者latest?),我們用概率決定把哪一個作為建議。我們從跟原始詞w相關(guān)的所有可能的正確拼寫中找到可能性最大的那個拼寫建議c:
argmaxc P(c|w)
通過貝葉斯定理,上式可以轉(zhuǎn)化為
argmaxc P(w|c) P(c) / P(w)
下面介紹一下上式中的含義:
P(c|w)代表在輸入單詞w 的情況下,你本來想輸入 單詞c的概率。
P(w|c)代表用戶想輸入單詞c卻輸入w的概率,這個可以我們認(rèn)為給定的。
P(c)代表在樣本數(shù)據(jù)中單詞c出現(xiàn)的概率
P(w)代表在樣本數(shù)字中單詞w出現(xiàn)的概率可以確定P(w)對于所有可能的單詞c概率都是一樣的,所以上式可以轉(zhuǎn)換為
argmaxc P(w|c) P(c)
我們所有的代碼都是基于這個公式來的,下面分析具體代碼實現(xiàn)
代碼分析
利用words()函數(shù)提取big.txt中的單詞
def words(text): return re.findall('[a-z]+', text.lower())
re.findall(‘[a-z]+’是利用python正則表達式模塊,提取所有的符合’[a-z]+’條件的,也就是由字母組成的單詞。(這里不詳細(xì)介紹正則表達式了,有興趣的同學(xué)可以看正則表達式簡介。text.lower()是將文本轉(zhuǎn)化為小寫字母,也就是“the”和“The”一樣定義為同一個單詞。
利用train()函數(shù)計算每個單詞出現(xiàn)的次數(shù)然后訓(xùn)練出一個合適的模型
def train(features):
model = collections.defaultdict(lambda: 1)
for f in features:
model[f] += 1
return model
NWORDS = train(words(file('big.txt').read()))
這樣NWORDS[w]代表了單詞w在樣本中出現(xiàn)的次數(shù)。如果有一個單詞并沒有出現(xiàn)在我們的樣本中該怎么辦?處理方法是將他們的次數(shù)默認(rèn)設(shè)為1,這里通過collections模塊和lambda表達式實現(xiàn)。
collections.defaultdict()創(chuàng)建了一個默認(rèn)的字典,lambda:1將這個字典中的每個值都默認(rèn)設(shè)為1。(lambda表達式可以看lambda簡介)
現(xiàn)在我們處理完了公式argmaxc P(w|c) P(c)中的P(c),接下來處理P(w|c)即想輸入單詞c卻錯誤地輸入單詞w的概率,通過 “edit distance“--將一個單詞變?yōu)榱硪粋€單詞所需要的編輯次數(shù)來衡量,一次edit可能是一次刪除,一個交換(兩個相鄰的字母),一次插入,一次修改。下面的函數(shù)返回一個將c進行一次編輯所有可能得到的單詞w的集合:
def edits1(word):
splits = [(word[:i], word[i:]) for i in range(len(word) + 1)]
deletes = [a + b[1:] for a, b in splits if b]
transposes = [a + b[1] + b[0] + b[2:] for a, b in splits if len(b)>1]
replaces = [a + c + b[1:] for a, b in splits for c in alphabet if b]
inserts = [a + c + b for a, b in splits for c in alphabet]
return set(deletes + transposes + replaces + inserts)
相關(guān)論文顯示,80-95%的拼寫錯誤跟想要拼寫的單詞都只有1個編輯距離,如果覺得一次編輯不夠,那我們再來一次
def known_edits2(word):
return set(e2 for e1 in edits1(word) for e2 in edits1(e1) if e2 in NWORDS)
同時還可能有編輯距離為0次的即本身就拼寫正確的:
def known(words):
return set(w for w in words if w in NWORDS)
我們假設(shè)編輯距離1次的概率遠(yuǎn)大于2次的,0次的遠(yuǎn)大于1次的。下面通過correct函數(shù)先選擇編輯距離最小的單詞,其對應(yīng)的P(w|c)就會越大,作為候選單詞,再選擇P(c)最大的那個單詞作為拼寫建議
def correct(word):
candidates = known([word]) or known(edits1(word)) or known_edits2(word) or [word]
return max(candidates, key=NWORDS.get)
-
代碼
+關(guān)注
關(guān)注
30文章
4807瀏覽量
68806 -
貝葉斯
+關(guān)注
關(guān)注
0文章
77瀏覽量
12582 -
python
+關(guān)注
關(guān)注
56文章
4801瀏覽量
84855
原文標(biāo)題:python實現(xiàn)拼寫檢查器21行輕松搞定
文章出處:【微信號:magedu-Linux,微信公眾號:馬哥Linux運維】歡迎添加關(guān)注!文章轉(zhuǎn)載請注明出處。
發(fā)布評論請先 登錄
相關(guān)推薦
分享50條經(jīng)典的Python一行代碼
用python編寫一個高效搜索代碼工具
如何實現(xiàn)計算機視覺的目標(biāo)檢測10行Python代碼幫你實現(xiàn)
如何使用50行Python代碼實現(xiàn)AI的動作平衡
盤點10個一行強大的、有趣的Python源代碼
python設(shè)計一個簡單推薦系統(tǒng)的資料和完整代碼
3行代碼實現(xiàn)單片機Uart功能
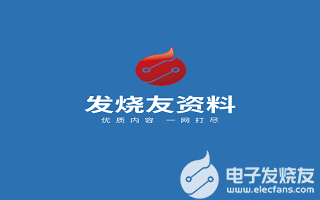
一行代碼將Python程序轉(zhuǎn)換為GUI應(yīng)用程序
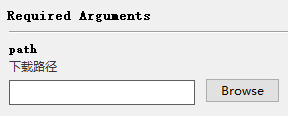
一行代碼將Python程序轉(zhuǎn)換為圖形界面應(yīng)用
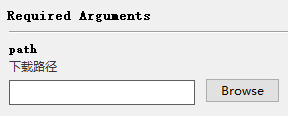
評論