使用scikit-learn進(jìn)行文本分類
多標(biāo)簽分類格式
對(duì)于多標(biāo)簽分類問(wèn)題而言,一個(gè)樣本可能同時(shí)屬于多個(gè)類別。如一個(gè)新聞屬于多個(gè)話題。這種情況下,因變量yy需要使用一個(gè)矩陣表達(dá)出來(lái)。
而多類別分類指的是y的可能取值大于2,但是y所屬類別是唯一的。它與多標(biāo)簽分類問(wèn)題是有嚴(yán)格區(qū)別的。所有的scikit-learn分類器都是默認(rèn)支持多類別分類的。但是,當(dāng)你需要自己修改算法的時(shí)候,也是可以使用scikit-learn實(shí)現(xiàn)多類別分類的前期數(shù)據(jù)準(zhǔn)備的。
多類別或多標(biāo)簽分類問(wèn)題,有兩種構(gòu)建分類器的策略:One-vs-All及One-vs-One。下面,通過(guò)一些例子進(jìn)行演示如何實(shí)現(xiàn)這兩類策略。
#from sklearn.preprocessing import MultiLabelBinarizery = [[2,3,4],[2],[0,1,3],[0,1,2,3,4],[0,1,2]]MultiLabelBinarizer().fit_transform(y)array([[0, 0, 1, 1, 1], [0, 0, 1, 0, 0], [1, 1, 0, 1, 0], [1, 1, 1, 1, 1], [1, 1, 1, 0, 0]])One-Vs-The-Rest策略
這個(gè)策略同時(shí)也稱為One-vs-all策略,即通過(guò)構(gòu)造K個(gè)判別式(K為類別的個(gè)數(shù)),第ii個(gè)判別式將樣本歸為第ii個(gè)類別或非第ii個(gè)類別。這種分類方法雖然比較耗時(shí)間,但是能夠通過(guò)每個(gè)類別對(duì)應(yīng)的判別式獲得關(guān)于該類別的直觀理解(如文本分類中每個(gè)話題可以通過(guò)只屬于該類別的高頻特征詞區(qū)分)。
多類別分類學(xué)習(xí)
from sklearn import datasetsfrom sklearn.multiclass import OneVsRestClassifierfrom sklearn.svm import LinearSVCiris = datasets.load_iris()X,y = iris.data,iris.targetOneVsRestClassifier(LinearSVC(random_state = 0)).fit(X,y).predict(X)array([0, 0, 0, 0, 0, 0, 0, 0, 0, 0, 0, 0, 0, 0, 0, 0, 0, 0, 0, 0, 0, 0, 0, 0, 0, 0, 0, 0, 0, 0, 0, 0, 0, 0, 0, 0, 0, 0, 0, 0, 0, 0, 0, 0, 0, 0, 0, 0, 0, 0, 1, 1, 1, 1, 1, 1, 1, 1, 1, 1, 1, 1, 1, 1, 1, 1, 1, 1, 1, 1, 2, 1, 1, 1, 1, 1, 1, 1, 1, 1, 1, 1, 1, 2, 2, 1, 1, 1, 1, 1, 1, 1, 1, 1, 1, 1, 1, 1, 1, 1, 2, 2, 2, 2, 2, 2, 2, 2, 2, 2, 2, 2, 2, 2, 2, 2, 2, 2, 2, 2, 2, 2, 2, 2, 2, 2, 2, 2, 2, 1, 2, 2, 2, 1, 2, 2, 2, 2, 2, 2, 2, 2, 2, 2, 2, 2, 2, 2, 2, 2])
多標(biāo)簽分類學(xué)習(xí)
Kaggle上有一個(gè)關(guān)于多標(biāo)簽分類問(wèn)題的競(jìng)賽:Multi-label classification of printed media articles to topics(地址:https://www.kaggle.com/c/wise-2014)。
關(guān)于該競(jìng)賽的介紹如下:
This is a multi-label classification competition for articles coming from Greek printed media. Raw data comes from the scanning of print media, article segmentation, and optical character segmentation, and therefore is quite noisy. Each article is examined by a human annotator and categorized to one or more of the topics being monitored. Topics range from specific persons, products, and companies that can be easily categorized based on keywords, to more general semantic concepts, such as environment or economy. Building multi-label classifiers for the automated annotation of articles into topics can support the work of human annotators by suggesting a list of all topics by order of relevance, or even automate the annotation process for media and/or categories that are easier to predict. This saves valuable time and allows a media monitoring company to expand the portfolio of media being monitored.
我們從該網(wǎng)站下載相應(yīng)的數(shù)據(jù),作為多標(biāo)簽分類的案例學(xué)習(xí)。
數(shù)據(jù)描述
這個(gè)文本數(shù)據(jù)集已經(jīng)用詞袋模型進(jìn)行形式化表示,共201561個(gè)特征詞,每個(gè)文本對(duì)應(yīng)一個(gè)或多個(gè)標(biāo)簽,共203個(gè)分類標(biāo)簽。該網(wǎng)站提供了兩種數(shù)據(jù)格式:ARFF和LIBSVM,ARFF格式的數(shù)據(jù)主要適用于weka,而LIBSVM格式適用于matlab中的LIBSVM模塊。這里,我們采用LIBSVM格式的數(shù)據(jù)。
數(shù)據(jù)的每一行以逗號(hào)分隔的整數(shù)序列開(kāi)頭,代表類別標(biāo)簽。緊接著是以\t分隔的id:value對(duì)。其中,id為特征詞的ID,value為特征詞在該文檔中的TF-IDF值。
形式如下。
58,152 833:0.032582 1123:0.003157 1629:0.038548 ...
數(shù)據(jù)載入
# load modulesimport os import sysimport numpy as npfrom sklearn.datasets import load_svmlight_filefrom sklearn.preprocessing import LabelBinarizerfrom sklearn.preprocessing import MultiLabelBinarizerfrom sklearn.linear_model import LogisticRegressionfrom sklearn.multiclass import OneVsRestClassifierfrom sklearn import metrics# set working directoryos.chdir("D:\\my_python_workfile\\Thesis\\kaggle_multilabel_classification")# read filesX_train,y_train = load_svmlight_file("./data/wise2014-train.libsvm",dtype=np.float64,multilabel=True)X_test,y_test = load_svmlight_file("./data/wise2014-test.libsvm",dtype = np.float64,multilabel=True)
模型擬合及預(yù)測(cè)
# transform y into a matrixmb = MultiLabelBinarizer()y_train = mb.fit_transform(y_train)# fit the model and predictclf = OneVsRestClassifier(LogisticRegression(),n_jobs=-1)clf.fit(X_train,y_train)pred_y = clf.predict(X_test)
模型評(píng)估
由于沒(méi)有關(guān)于測(cè)試集的真實(shí)標(biāo)簽,這里看看訓(xùn)練集的預(yù)測(cè)情況。
# training set resulty_predicted = clf.predict(X_train)#report #print(metrics.classification_report(y_train,y_predicted))import numpy as npnp.mean(y_predicted == y_train)0.99604661023482433
保存結(jié)果
# write the outputout_file = open("pred.csv","w")out_file.write("ArticleId,Labels\n")id = 64858for i in xrange(pred_y.shape[0]): label = list(mb.classes_[np.where(pred_y[i,:]==1)[0]].astype("int")) label = " ".join(map(str,label)) if label == "": # if the label is empty label = "103" out_file.write(str(id+i)+","+label+"\n")out_file.close()One-Vs-One策略
One-Vs-One策略即是兩兩類別之間建立一個(gè)判別式,這樣,總共需要K(K?1)/2K(K?1)/2個(gè)判別式,最后通過(guò)投票的方式確定樣本所屬類別。
多類別分類學(xué)習(xí)
from sklearn import datasetsfrom sklearn.multiclass import OneVsOneClassifierfrom sklearn.svm import LinearSVCiris = datasets.load_iris()X,y = iris.data,iris.targetOneVsOneClassifier(LinearSVC(random_state = 0)).fit(X,y).predict(X)array([0, 0, 0, 0, 0, 0, 0, 0, 0, 0, 0, 0, 0, 0, 0, 0, 0, 0, 0, 0, 0, 0, 0, 0, 0, 0, 0, 0, 0, 0, 0, 0, 0, 0, 0, 0, 0, 0, 0, 0, 0, 0, 0, 0, 0, 0, 0, 0, 0, 0, 1, 1, 1, 1, 1, 1, 1, 1, 1, 1, 1, 1, 1, 1, 1, 1, 1, 1, 1, 1, 2, 1, 2, 1, 1, 1, 1, 1, 1, 1, 1, 1, 1, 2, 1, 1, 1, 1, 1, 1, 1, 1, 1, 1, 1, 1, 1, 1, 1, 1, 2, 2, 2, 2, 2, 2, 2, 2, 2, 2, 2, 2, 2, 2, 2, 2, 2, 2, 2, 2, 2, 2, 2, 2, 2, 2, 2, 2, 2, 2, 2, 2, 2, 2, 2, 2, 2, 2, 2, 2, 2, 2, 2, 2, 2, 2, 2, 2, 2, 2])參考文獻(xiàn)
-
文本分類
+關(guān)注
關(guān)注
0文章
18瀏覽量
7331
原文標(biāo)題:使用scikit-learn實(shí)現(xiàn)多類別及多標(biāo)簽分類算法
文章出處:【微信號(hào):AI_shequ,微信公眾號(hào):人工智能愛(ài)好者社區(qū)】歡迎添加關(guān)注!文章轉(zhuǎn)載請(qǐng)注明出處。
發(fā)布評(píng)論請(qǐng)先 登錄
相關(guān)推薦
Python機(jī)器學(xué)習(xí)庫(kù)談Scikit-learn技術(shù)
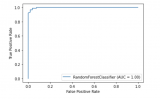
通用Python機(jī)器學(xué)習(xí)庫(kù)scikit-learn
NLPIR平臺(tái)在文本分類方面的技術(shù)解析
基于Python的scikit-learn編程實(shí)例
scikit-learn K近鄰法類庫(kù)使用的經(jīng)驗(yàn)總結(jié)
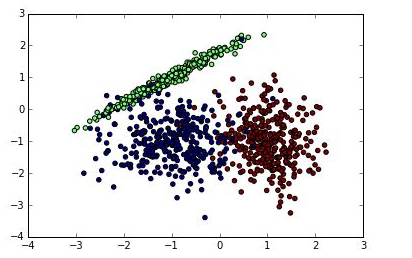
textCNN論文與原理——短文本分類
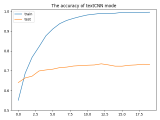
基于Python的scikit-learn包實(shí)現(xiàn)機(jī)器學(xué)習(xí)
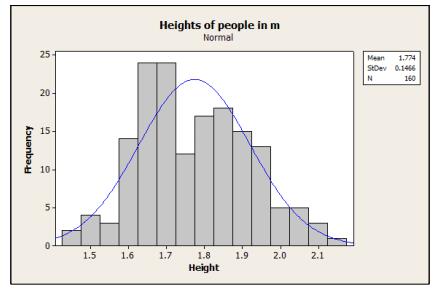
評(píng)論