在本文中,我們將介紹如何在PyCharm中訓練數據集。PyCharm是一款流行的Python集成開發(fā)環(huán)境,提供了許多用于數據科學和機器學習的工具。
1. 安裝PyCharm和相關庫
首先,確保你已經安裝了PyCharm。接下來,你需要安裝一些用于數據處理和機器學習的庫。在PyCharm中,你可以通過以下步驟安裝庫:
- 打開PyCharm,創(chuàng)建一個新的項目。
- 轉到“File” > “Settings”(或“PyCharm” > “Preferences”在Mac上)。
- 在“Project: [Your Project Name]”下,選擇“Project Interpreter”。
- 點擊“+”號添加新的庫。你可以搜索并安裝以下庫:
- NumPy
- Pandas
- Matplotlib
- Scikit-learn
- TensorFlow 或 PyTorch(根據你的需要選擇)
2. 數據預處理
數據預處理是機器學習中非常重要的一步。在PyCharm中,你可以使用Pandas庫來處理數據。
2.1 導入數據
假設你有一個CSV文件,你可以使用Pandas的read_csv
函數來導入數據:
import pandas as pd
data = pd.read_csv('your_dataset.csv')
2.2 數據清洗
數據清洗包括處理缺失值、異常值和重復數據。
- 處理缺失值 :可以使用
fillna
或dropna
方法。
data.fillna(method='ffill', inplace=True) # 前向填充
data.dropna(inplace=True) # 刪除缺失值
- 處理異常值 :可以使用箱型圖(IQR)方法。
Q1 = data.quantile(0.25)
Q3 = data.quantile(0.75)
IQR = Q3 - Q1
data = data[~((data < (Q1 - 1.5 * IQR)) |(data > (Q3 + 1.5 * IQR))).any(axis=1)]
- 刪除重復數據 :
data.drop_duplicates(inplace=True)
2.3 特征工程
特征工程是創(chuàng)建新特征或修改現有特征以提高模型性能的過程。
- 特征選擇 :可以使用相關性分析、遞歸特征消除等方法。
correlation_matrix = data.corr()
important_features = correlation_matrix.index[abs(correlation_matrix["target"]) > 0.5]
- 特征轉換 :可以使用Pandas的
apply
方法或Scikit-learn的Transformers
。
def transform_feature(x):
# 你的轉換邏輯
return transformed_value
data['new_feature'] = data['existing_feature'].apply(transform_feature)
3. 模型選擇
在PyCharm中,你可以使用Scikit-learn庫來選擇和訓練模型。
3.1 劃分數據集
使用train_test_split
函數將數據集劃分為訓練集和測試集。
from sklearn.model_selection import train_test_split
X = data.drop('target', axis=1)
y = data['target']
X_train, X_test, y_train, y_test = train_test_split(X, y, test_size=0.2, random_state=42)
3.2 選擇模型
Scikit-learn提供了許多內置模型,如線性回歸、決策樹、隨機森林等。你可以根據問題的性質選擇合適的模型。
from sklearn.ensemble import RandomForestClassifier
model = RandomForestClassifier()
3.3 訓練模型
使用訓練集數據訓練模型。
model.fit(X_train, y_train)
4. 模型評估
評估模型的性能,可以使用準確率、召回率、F1分數等指標。
from sklearn.metrics import accuracy_score, classification_report
y_pred = model.predict(X_test)
print("Accuracy:", accuracy_score(y_test, y_pred))
print(classification_report(y_test, y_pred))
5. 模型優(yōu)化
使用交叉驗證、超參數調優(yōu)等方法來優(yōu)化模型。
5.1 交叉驗證
使用cross_val_score
函數進行交叉驗證。
from sklearn.model_selection import cross_val_score
scores = cross_val_score(model, X, y, cv=5)
print("Cross-validated scores:", scores)
-
數據處理
+關注
關注
0文章
622瀏覽量
28692 -
機器學習
+關注
關注
66文章
8458瀏覽量
133285 -
數據集
+關注
關注
4文章
1212瀏覽量
24940
發(fā)布評論請先 登錄
相關推薦
Pycharm安裝方法
pycharm的安裝使用教程
人工智能訓練數據集:基礎與發(fā)展
人工智能訓練數據集:誤區(qū)、挑戰(zhàn)與應對方法
PyTorch教程15.9之預訓練BERT的數據集
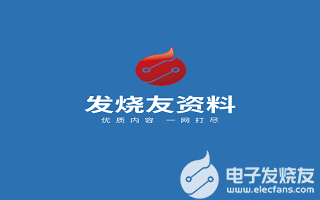
評論