what are tensorcores
TensorCore是一個(gè)硬件概念,主要是用于加速矩陣乘操作運(yùn)算(我們也叫MMA,Matrix Multiply Add),執(zhí)行的是:
D = A * B + C
同時(shí)也支持多種輸入類型,數(shù)值累加類型。
編程層次上,TensorCore處于Warp(連續(xù)的32個(gè)threads)這一層,一個(gè)WARP內(nèi)持有A, B, C, D四個(gè)操作數(shù)的數(shù)據(jù)。
上圖是Ampere架構(gòu)支持的MMA指令,支持多種尺寸,數(shù)據(jù)類型。
Slides下面就是介紹各種尺寸的MMA,我們可以結(jié)合代碼跑一下
S8 * S8 + S32 Code
使用TensorCore的時(shí)候,對數(shù)據(jù)排布是有特殊要求的。MMA指令是在一個(gè)WARP內(nèi)執(zhí)行,所以各個(gè)線程對應(yīng)取數(shù)據(jù)的位置也是有特殊的映射關(guān)系。
首先來個(gè)簡單的 int8 x int8 = int32 的(8x16 matmul 16x8 = 8x8)運(yùn)算,Slides里的排布是這樣:
每個(gè)線程持有 A的4x8bit = 32bit 數(shù)據(jù),B的4x8bit = 32bit 數(shù)據(jù),C/D的 2x32bit = 64bit 數(shù)據(jù)
我們假設(shè)使用的矩陣為:
我們把線程映射跟元素寫到一塊:
而由于tensor core instruction is TN layout.
這里還是沿用blas計(jì)算庫的說法,blas庫里,會將 a x b = c -> b_T x a_T = c_T,這里的T說的是B矩陣是transpose的,也即A矩陣是RowMajor, B矩陣是ColMajor.
所以實(shí)際上應(yīng)該是:
可以看到跟A矩陣是完全一樣了,后面取元素的時(shí)候兩個(gè)矩陣寄存器所使用的index是一致的
這里使用的代碼是slides里的example。
先簡單寫個(gè)初始化的kernel:
#include"stdio.h" #include"stdint.h" __global__voidset_value(int8_t*x,int32_telem_cnt){ for(inti=0;i(i%8); } }
接下來是TensorCore運(yùn)算的kernel,需要注意的是這里用的都是int32類型,而我們執(zhí)行的是 s8 x s8 = s32 的計(jì)算,調(diào)用的時(shí)候需要reinterpret_cast下。
//DoAxB+C=D. __global__voidtensor_core_example_8x8x16(int32_t*D, uint32_tconst*A, uint32_tconst*B, int32_tconst*C){ //ComputethecoordinatesofaccessestoAandBmatrices intouter=threadIdx.x/4;//morndimension intinner=threadIdx.x%4;//kdimension //Computethecoordinatesfortheaccumulatormatrices intc_row=threadIdx.x/4; intc_col=2*(threadIdx.x%4); //Computelinearoffsetsintoeachmatrix intab_idx=outer*4+inner; intcd_idx=c_row*8+c_col; //IssueTensorCoreoperation asmvolatile("mma.sync.aligned.m8n8k16.row.col.s32.s8.s8.s32{%0,%1},{%2},{%3},{%4,%5}; " :"=r"(D[cd_idx]),"=r"(D[cd_idx+1]) :"r"(A[ab_idx]),"r"(B[ab_idx]),"r"(C[cd_idx]),"r"(C[cd_idx+1])); } 最后打印輸出結(jié)果:
__global__voidprintMatrix(int32_t*result,constintm,constintn){ for(introw=0;row>>(a,m*k); set_value<<<1,?1>>>(b,k*n); cudaMemset(c,0,sizeof(int32_t)*m*n); cudaMemset(d,0,sizeof(int32_t)*m*n); tensor_core_example_8x8x16<<<1,?32>>>(reinterpret_cast(d), reinterpret_cast (a), reinterpret_cast (b), reinterpret_cast (c)); printMatrix<<<1,?1>>>(d,m,n); cudaDeviceSynchronize(); cudaFree(a); cudaFree(b); cudaFree(c); cudaFree(d); }
舉一反三
下面我們也可以舉一反三,寫下 f16*f16+fp32的 tensorcore程序,對應(yīng)的指令是 16 x 8 x 8,不過線程持有的數(shù)據(jù)跟前面的例子有些不同,需要改下
#include"stdio.h" #include"stdint.h" #include"cuda_fp16.h" template__global__voidset_value(T*x,int32_telem_cnt){ for(inti=0;i(i%8); } } __global__voidtensor_core_example_16x8x8(float*D, uint32_tconst*A, uint32_tconst*B, floatconst*C){ //ComputethecoordinatesofaccessestoAandBmatrices intouter=threadIdx.x/4;//morndimension intinner=threadIdx.x%4;//kdimension //Computethecoordinatesfortheaccumulatormatrices intc_row=threadIdx.x/4; intc_col=2*(threadIdx.x%4); //Computelinearoffsetsintoeachmatrix intab_idx=outer*4+inner; intcd_idx=c_row*8+c_col; //IssueTensorCoreoperation asmvolatile("mma.sync.aligned.m16n8k8.row.col.f32.f16.f16.f32{%0,%1,%2,%3},{%4,%5},{%6},{%7,%8,%9,%10}; " :"=f"(D[cd_idx]),"=f"(D[cd_idx+1]),"=f"(D[cd_idx+64]),"=f"(D[cd_idx+1+64]) : "r"(A[ab_idx]),"r"(A[ab_idx+32]), "r"(B[ab_idx]), "f"(C[cd_idx]),"f"(C[cd_idx+1]),"f"(C[cd_idx+64]),"f"(C[cd_idx+1+64]) ); } __global__voidprintMatrix(float*result,constintm,constintn){ for(introw=0;row(result[row*n+col])); } printf(" "); } } intmain(){ half*a; half*b; float*c; float*d; constint32_tm=16; constint32_tk=8; constint32_tn=8; cudaMalloc(&a,m*k*sizeof(half)); cudaMalloc(&b,k*n*sizeof(half)); cudaMalloc(&c,m*n*sizeof(float)); cudaMalloc(&d,m*n*sizeof(float)); set_value <<<1,?1>>>(a,m*k); set_value <<<1,?1>>>(b,k*n); cudaMemset(c,0,sizeof(float)*m*n); cudaMemset(d,0,sizeof(float)*m*n); tensor_core_example_16x8x8<<<1,?32>>>(reinterpret_cast (d), reinterpret_cast (a), reinterpret_cast (b), reinterpret_cast (c)); printMatrix<<<1,?1>>>(d,m,n); cudaDeviceSynchronize(); cudaFree(a); cudaFree(b); cudaFree(c); cudaFree(d); }
可以看到不同的MMA指令會對應(yīng)不同的矩陣規(guī)模,不同的數(shù)據(jù)類型。在CUTLASS,上述的這些MMA被統(tǒng)一到一個(gè)模板里:
實(shí)際使用的話,只需對應(yīng)實(shí)例化MMA模板即可:
DATA Movement
下面幾張Slides談?wù)摰氖蔷仃嚦酥袛?shù)據(jù)搬運(yùn)的部分,以及新架構(gòu)引入的LDMatrix指令。
這張Slide還是以S8 x S8 + S32的mma為例,前面我們也推導(dǎo)過,一個(gè)WARP完成 8x16 matmul 16x8, 那么一個(gè)WARP加載A矩陣和B矩陣一共需要 (8x16 + 16x8) = 256B,F(xiàn)LOPS計(jì)算如下:
C矩陣一共8*8=64個(gè)元素 每個(gè)元素需要16次乘法和加法, FLOPS=64*16*2=2048
兩者一除得到計(jì)算訪存比為 8flops/byte。
那么我們再看下Ampere架構(gòu)白皮書里面標(biāo)注的設(shè)計(jì)規(guī)格,A100的Int8 tensorcore算力是624TFLOPS(312是FP16,int8對應(yīng)翻一倍),80GB A100的HBM速度為1.6TB/s,那么其理想計(jì)算訪存比是 400flops/byte
相較兩者訪存比,可以看到使用了TensorCore后,訪存成為了瓶頸,這也是為什么數(shù)據(jù)搬運(yùn)在優(yōu)化GEMM里是很重要的一環(huán)。
這里我覺得是作為一種理想情況的估算,實(shí)際情況可能更復(fù)雜,需要考慮緩存命中率等(參考知乎李少俠的文章)
因此cutlass抽象了一套高效的數(shù)據(jù)搬運(yùn)流程,過往很多GEMM優(yōu)化文章都有介紹,就不贅述了:
其中在Ampere架構(gòu)里面,新引入了AsyncCopy機(jī)制,也就是在Global Memory 到 SharedMemory 這一個(gè)環(huán)節(jié)。以往我們需要從Global Memory讀取到線程寄存器,再從寄存器里存儲到SharedMemory,但有了這個(gè)指令后,我們可以一步到位,從GlobalMemory -> SharedMemory,一定程度減輕了寄存器壓力。(如果你常profile GEMM應(yīng)該能有所體會)
并且它是一種異步操作,意味著我們可以提前發(fā)射出好幾輪(在cutlass里往往稱為Stage)數(shù)據(jù)預(yù)取的指令,以實(shí)現(xiàn)延遲隱藏(我搬我的,你算你的)。
而另外一個(gè)比較特殊的指令則是LDMatrix,這個(gè)指令是用在SharedMemory到Register的過程。
為了盡可能打滿帶寬,在GlobalMemory->SharedMemory這一環(huán)節(jié)中,每個(gè)線程都是以128bit的訪問粒度去存儲。而前面也提到TensorCore對應(yīng)每個(gè)線程對數(shù)據(jù)有不同的索引,這也就導(dǎo)致每個(gè)線程需要的元素在SharedMemory上是不連續(xù)的。
以Slides為例,我們看T0線程,它需要T0,T8,T16,T24對應(yīng)SharedMemory的第一個(gè)元素。在沒有LDMatrix之前,它需要對應(yīng)四次LDS32操作,而如果我們調(diào)用LDMatrix,可以一個(gè)指令就完成上述的操作:
下面我們簡單提一下Cutlass的crosswise Layout(我看的不是很明白)。通常來說為了避免BankConflict,我們常見的做法是Padding多一個(gè)元素,讓W(xué)arp內(nèi)線程訪問錯(cuò)開,但是這樣肯定是帶來了SharedMemory浪費(fèi)。而Cutlass提出了一種新的Layout,通過一系列很復(fù)雜的異或操作算出來了一個(gè)索引,最終大概長這樣:
這里每個(gè)線程存了128bit數(shù)據(jù),也就是占了4個(gè)bank。還是以剛剛線程0所需的數(shù)據(jù)為例,可以看到T0 T8 T16 T24都是錯(cuò)開到不同的Bank上(其他線程同理)
下面是一個(gè)LDMatrix的example
PS:我不知道我寫的對不對,至少從結(jié)果上看還挺合理,如果有錯(cuò)也麻煩指正
LDMatrix example
#include"stdio.h" #include"stdint.h" #include"cuda_fp16.h" #defineLDMATRIX_X4(R0,R1,R2,R3,addr) asmvolatile("ldmatrix.sync.aligned.x4.m8n8.shared.b16{%0,%1,%2,%3},[%4]; " :"=r"(R0),"=r"(R1),"=r"(R2),"=r"(R3) :"r"(addr)) template__global__voidset_value(T*x,int32_telem_cnt){ for(inti=0;i(i%8); } } //從CUTLASS里抄的 __device__uint32_tcast_smem_ptr_to_uint(voidconst*constptr){ //WeprefertousethenewCVTAintrinsicsiftheyareavailable,otherwisewewillfallbackto //thepreviousinternalintrinsicsiftheyareavailable. #ifCUTE_CVTA_GENERIC_TO_SHARED_ACTIVATED // //ThisNVVMintrinsicconvertsanaddressinsharedmemorytoaplain //unsignedinteger.Thisisnecessarytopasstosharedmemoryinstructions //ininlinePTX. // //InCUDA11andbeyond,thisreplaces__nvvm_get_smem_pointer()[onlyavailablein10.2]. // //__device__size_t__cvta_generic_to_shared(void*ptr); ///CUTEhelpertogetSMEMpointer returnstatic_cast (__cvta_generic_to_shared(ptr)); #elifCUTE_NVVM_GET_SMEM_POINTER_ACTIVATED return__nvvm_get_smem_pointer(ptr); #elifdefined(__CUDA_ARCH__) uint32_tsmem_ptr; asm( "{.reg.u64smem_ptr;cvta.to.shared.u64smem_ptr,%1;cvt.u32.u64%0,smem_ptr;} " :"=r"(smem_ptr):"l"(ptr)); returnsmem_ptr; #else (void)ptr; printf("ERROR:cast_smem_ptr_to_uintnotsupportedbutused. "); return0; #endif } __global__voidldmatrix_example(uint32_t*x, uint32_t*y){ constint32_trow_tid=threadIdx.x/8; constint32_tcol_tid=threadIdx.x%8; uint32_tRegisterLoad[4]; uint32_tRegisterTensorcore[4]; __shared__halfsmem[4][64]; *reinterpret_cast (RegisterLoad)=*reinterpret_cast ((x+threadIdx.x*4)); half*half_register_load_ptr=reinterpret_cast (RegisterLoad); if(threadIdx.x==0){ printf("ThreadIdx:%d,Valueis:%f,%f,%f,%f,%f,%f,%f,%f. ",threadIdx.x, static_cast (half_register_load_ptr[0]),static_cast (half_register_load_ptr[1]), static_cast (half_register_load_ptr[2]),static_cast (half_register_load_ptr[3]), static_cast (half_register_load_ptr[4]),static_cast (half_register_load_ptr[5]), static_cast (half_register_load_ptr[6]),static_cast (half_register_load_ptr[7])); } int32_txor_idx=threadIdx.x; if(row_tid==1){ xor_idx^=1; } if(row_tid==2){ xor_idx^=2; } if(row_tid==3){ xor_idx^=3; } constint32_tstore_smem_row_tid=xor_idx/8; constint32_tstore_smem_col_tid=xor_idx%8; //if(threadIdx.x==0){ printf("ThreadIdx:%d,XorIdxis:%d,store_smem_row_tidis:%d,store_smem_col_tidis:%d. ",threadIdx.x,xor_idx,store_smem_row_tid,store_smem_col_tid*8); //} half*smem_ptr=&(smem[store_smem_row_tid][store_smem_col_tid*8]);//smem[store_smem_row_tid][store_smem_col_tid*4]; *reinterpret_cast (smem_ptr)=*reinterpret_cast (RegisterLoad); __syncthreads(); if(threadIdx.x==0||threadIdx.x==8||threadIdx.x==16||threadIdx.x==24){ printf("ThreadIdx:%d,SMEMValueis:%f,%f,%f,%f,%f,%f,%f,%f. ",threadIdx.x, static_cast (smem[0][0]),static_cast (smem[0][1]), static_cast (smem[0][2]),static_cast (smem[0][3]), static_cast (smem[0][4]),static_cast (smem[0][5]), static_cast (smem[0][6]),static_cast (smem[0][7])); } uint32_taddr=cast_smem_ptr_to_uint(smem_ptr); LDMATRIX_X4(RegisterTensorcore[0],RegisterTensorcore[1],RegisterTensorcore[2],RegisterTensorcore[3],addr); half*half_register_tensorcore_ptr=reinterpret_cast (RegisterTensorcore); if(threadIdx.x==0){ printf("AfterLDMATRIX,ThreadIdx:%d,Valueis:%f,%f,%f,%f,%f,%f,%f,%f. ", threadIdx.x, static_cast (half_register_tensorcore_ptr[0]),static_cast (half_register_tensorcore_ptr[1]), static_cast (half_register_tensorcore_ptr[2]),static_cast (half_register_tensorcore_ptr[3]), static_cast (half_register_tensorcore_ptr[4]),static_cast (half_register_tensorcore_ptr[5]), static_cast (half_register_tensorcore_ptr[6]),static_cast (half_register_tensorcore_ptr[7])); } } __global__voidprintMatrix(half*result,constintm,constintn){ for(introw=0;row(result[row*n+col])); } printf(" "); } } intmain(){ half*x; half*y; constint32_tm=16; constint32_tk=16; constint32_tn=8; cudaMalloc(&x,m*k*sizeof(half)); cudaMalloc(&y,m*k*sizeof(half)); set_value <<<1,?1>>>(x,m*k); cudaMemset(y,0,sizeof(half)*m*k); ldmatrix_example<<<1,?32>>>(reinterpret_cast (x), reinterpret_cast (y)); //printMatrix<<<1,?1>>>(y,m,k); cudaDeviceSynchronize(); cudaFree(x); cudaFree(y); }
對于 cast_smem_ptr_to_uint 這個(gè)函數(shù)我也不是很清楚,我從元戎啟行的矩陣轉(zhuǎn)置Blog里摘了一段:
需要額外注意的是,共享內(nèi)存的地址并不是全局同步地址(GenericAddress),因此在使用共享內(nèi)存地址讀取或?qū)懭霐?shù)據(jù)前,要經(jīng)過一次內(nèi)置函數(shù)__cvta_generic_to_shared,當(dāng)然也可以自己手寫PTX
xor 換算索引 example
foriinrange(8,16): print(i,i^1) foriinrange(16,24): print(i,i^2) foriinrange(24,32): print(i,i^3)s 審核編輯:黃飛
-
寄存器
+關(guān)注
關(guān)注
31文章
5424瀏覽量
123446 -
數(shù)據(jù)類型
+關(guān)注
關(guān)注
0文章
237瀏覽量
13836 -
線程
+關(guān)注
關(guān)注
0文章
507瀏覽量
20085 -
Warp
+關(guān)注
關(guān)注
0文章
10瀏覽量
9651
原文標(biāo)題:亂談CUTLASS GTC2020 SLIDES
文章出處:【微信號:GiantPandaCV,微信公眾號:GiantPandaCV】歡迎添加關(guān)注!文章轉(zhuǎn)載請注明出處。
發(fā)布評論請先 登錄
KITA2GTC3325VTRBSTOBO1開發(fā)板運(yùn)行的是什么系統(tǒng)?
【限時(shí)領(lǐng)取精美禮品】報(bào)名2022 GTC大會,與行業(yè)大咖探索 AI 前沿科技
【中獎(jiǎng)公示】恭喜在GTC22直播間中獎(jiǎng)幸運(yùn)鵝~快來登記領(lǐng)獎(jiǎng)吧~
NVIDIA安培GPU或在明年3月底的GTC2020大會上推出
NVIDIA GTC或公布新一代Ampere安培架構(gòu)的GPU 將基于臺積電7nm工藝
英偉達(dá)將在GTC 2020至少展示6款機(jī)器人
NVIDIA GTC 2020大會如期舉行 官方表示將對場館進(jìn)行全面消毒
NVIDIA宣布暫時(shí)停止分享GTC 2020的相關(guān)新聞 下一代“安培”核心正式跳票
使用CUTLASS實(shí)現(xiàn)高性能矩陣乘法
MAX25400GTC/V+ MAX25400GTC/V+ - (Maxim Integrated) - 專用 IC
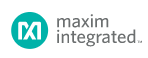
GTC23 | GTC 大會今日開幕!主題演講將于明日全球首播!
GTC 2023:阿里巴巴CUTLASS優(yōu)化探索推薦系統(tǒng)中的應(yīng)用
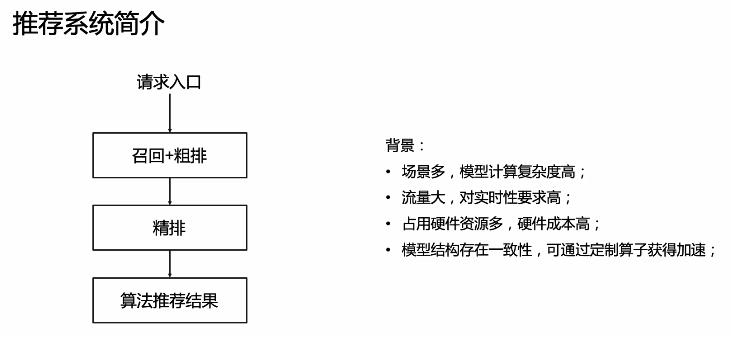
詳解CUTLASS的工作原理
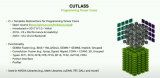
NVIDIA GTC 2025大會即將啟幕
NVIDIA GTC 2025精華一文讀完 黃仁勛在GTC上的主題演講
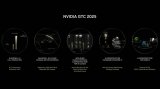
評論