前言
Hello大家好,今天給大家分享一下如何基于YOLOv8姿態(tài)評(píng)估模型,實(shí)現(xiàn)在自定義數(shù)據(jù)集上,完成自定義姿態(tài)評(píng)估模型的訓(xùn)練與推理。
01tiger-pose數(shù)據(jù)集
YOLOv8官方提供了一個(gè)自定義tiger-pose數(shù)據(jù)集(老虎姿態(tài)評(píng)估),總計(jì)數(shù)據(jù)有263張圖像、其中210張作為訓(xùn)練集、53張作為驗(yàn)證集。
其中YOLOv8-pose的數(shù)據(jù)格式如下:
解釋一下:
Class-index 表示對(duì)象類型索引,從0開始 后面的四個(gè)分別是對(duì)象的中心位置與寬高 xc、yc、width、height px1,py1表示第一個(gè)關(guān)鍵點(diǎn)坐標(biāo)、p1v表示師傅可見,默認(rèn)填2即可。 kpt_shape=12x2表示有12個(gè)關(guān)鍵點(diǎn),每個(gè)關(guān)鍵點(diǎn)是x,y
02模型訓(xùn)練
跟訓(xùn)練YOLOv8對(duì)象檢測(cè)模型類似,直接運(yùn)行下面的命令行即可:
yolotrainmodel=yolov8n-pose.ptdata=tiger_pose_dataset.yamlepochs=100imgsz=640batch=1
03模型導(dǎo)出預(yù)測(cè)
訓(xùn)練完成以后模型預(yù)測(cè)推理測(cè)試 使用下面的命令行:
yolo predict model=tiger_pose_best.pt source=D:/123.jpg
導(dǎo)出模型為ONNX格式,使用下面命令行即可
yolo export model=tiger_pose_best.pt format=onnx
04部署推理
基于ONNX格式模型,采用ONNXRUNTIME推理結(jié)果如下:
ORT相關(guān)的推理演示代碼如下:
def ort_pose_demo(): # initialize the onnxruntime session by loading model in CUDA support model_dir = "tiger_pose_best.onnx" session = onnxruntime.InferenceSession(model_dir, providers=['CUDAExecutionProvider']) # 就改這里, 把RTSP的地址配到這邊就好啦,然后直接運(yùn)行,其它任何地方都不準(zhǔn)改! # 切記把 yolov8-pose.onnx文件放到跟這個(gè)python文件同一個(gè)文件夾中! frame = cv.imread("D:/123.jpg") bgr = format_yolov8(frame) fh, fw, fc = frame.shape start = time.time() image = cv.dnn.blobFromImage(bgr, 1 / 255.0, (640, 640), swapRB=True, crop=False) # onnxruntime inference ort_inputs = {session.get_inputs()[0].name: image} res = session.run(None, ort_inputs)[0] # matrix transpose from 1x8x8400 => 8400x8 out_prob = np.squeeze(res, 0).T result_kypts, confidences, boxes = wrap_detection(bgr, out_prob) for (kpts, confidence, box) in zip(result_kypts, confidences, boxes): cv.rectangle(frame, box, (0, 0, 255), 2) cv.rectangle(frame, (box[0], box[1] - 20), (box[0] + box[2], box[1]), (0, 255, 255), -1) cv.putText(frame, ("%.2f" % confidence), (box[0], box[1] - 10), cv.FONT_HERSHEY_SIMPLEX, .5, (0, 0, 0)) cv.circle(frame, (int(kpts[0]), int(kpts[1])), 3, (255, 0, 255), 4, 8, 0) cv.circle(frame, (int(kpts[2]), int(kpts[3])), 3, (255, 0, 255), 4, 8, 0) cv.circle(frame, (int(kpts[4]), int(kpts[5])), 3, (255, 0, 255), 4, 8, 0) cv.circle(frame, (int(kpts[6]), int(kpts[7])), 3, (255, 0, 255), 4, 8, 0) cv.circle(frame, (int(kpts[8]), int(kpts[9])), 3, (255, 0, 255), 4, 8, 0) cv.circle(frame, (int(kpts[10]), int(kpts[11])), 3, (255, 0, 255), 4, 8, 0) cv.circle(frame, (int(kpts[12]), int(kpts[13])), 3, (255, 0, 255), 4, 8, 0) cv.circle(frame, (int(kpts[14]), int(kpts[15])), 3, (255, 0, 255), 4, 8, 0) cv.circle(frame, (int(kpts[16]), int(kpts[17])), 3, (255, 0, 255), 4, 8, 0) cv.circle(frame, (int(kpts[18]), int(kpts[19])), 3, (255, 0, 255), 4, 8, 0) cv.circle(frame, (int(kpts[20]), int(kpts[21])), 3, (255, 0, 255), 4, 8, 0) cv.circle(frame, (int(kpts[22]), int(kpts[23])), 3, (255, 0, 255), 4, 8, 0) cv.imshow("Tiger Pose Demo - gloomyfish", frame) cv.waitKey(0) cv.destroyAllWindows()
審核編輯:湯梓紅
-
模型
+關(guān)注
關(guān)注
1文章
3462瀏覽量
49785 -
數(shù)據(jù)集
+關(guān)注
關(guān)注
4文章
1220瀏覽量
25183 -
命令行
+關(guān)注
關(guān)注
0文章
80瀏覽量
10505
原文標(biāo)題:【YOLOv8】自定義姿態(tài)評(píng)估模型訓(xùn)練
文章出處:【微信號(hào):CVSCHOOL,微信公眾號(hào):OpenCV學(xué)堂】歡迎添加關(guān)注!文章轉(zhuǎn)載請(qǐng)注明出處。
發(fā)布評(píng)論請(qǐng)先 登錄
相關(guān)推薦
maixcam部署yolov5s 自定義模型
請(qǐng)問如何在imx8mplus上部署和運(yùn)行YOLOv5訓(xùn)練的模型?
YOLOv8自定義數(shù)據(jù)集訓(xùn)練到模型部署推理簡(jiǎn)析
TensorRT 8.6 C++開發(fā)環(huán)境配置與YOLOv8實(shí)例分割推理演示
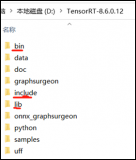
在AI愛克斯開發(fā)板上用OpenVINO?加速YOLOv8目標(biāo)檢測(cè)模型
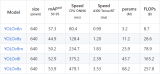
YOLOv8版本升級(jí)支持小目標(biāo)檢測(cè)與高分辨率圖像輸入
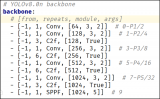
AI愛克斯開發(fā)板上使用OpenVINO加速YOLOv8目標(biāo)檢測(cè)模型
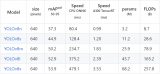
教你如何用兩行代碼搞定YOLOv8各種模型推理
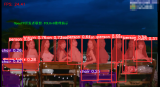
三種主流模型部署框架YOLOv8推理演示
YOLOv8實(shí)現(xiàn)任意目錄下命令行訓(xùn)練
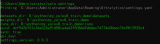
基于YOLOv8的自定義醫(yī)學(xué)圖像分割
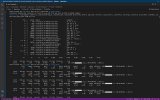
如何基于深度學(xué)習(xí)模型訓(xùn)練實(shí)現(xiàn)圓檢測(cè)與圓心位置預(yù)測(cè)
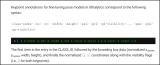
如何基于深度學(xué)習(xí)模型訓(xùn)練實(shí)現(xiàn)工件切割點(diǎn)位置預(yù)測(cè)
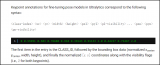
YOLOv8實(shí)現(xiàn)旋轉(zhuǎn)對(duì)象檢測(cè)
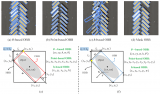
評(píng)論