前言
今天我們一起來看一下如何使用LabVIEW實(shí)現(xiàn)語義分割。
一、什么是語義分割
**圖像語義分割(semantic segmentation),從字面意思上理解就是讓計(jì)算機(jī)根據(jù)圖像的語義來進(jìn)行分割,例如讓計(jì)算機(jī)在輸入下面左圖的情況下,能夠輸出右圖。語義在語音識別中指的是語音的意思,在圖像領(lǐng)域,語義指的是圖像的內(nèi)容,對圖片意思的理解,比如下圖的語義就是一個(gè)人牽著四只羊;分割的意思是從像素的角度分割出圖片中的不同對象,對原圖中的每個(gè)像素都進(jìn)行標(biāo)注,比如下圖中淺黃色代表人,藍(lán)綠色代表羊。語義分割任務(wù)就是將圖片中的不同類別,用不同的顏色標(biāo)記出來,每一個(gè)類別使用一種顏色。常用于醫(yī)學(xué)圖像,衛(wèi)星圖像,無人車駕駛,機(jī)器人等領(lǐng)域。 **
- 如何做到將像素點(diǎn)上色呢?
**語義分割的輸出和圖像分類網(wǎng)絡(luò)類似,圖像分類類別數(shù)是一個(gè)一維的one hot 矩陣。例如:三分類的[0,1,0]。語義分割任務(wù)最后的輸出特征圖是一個(gè)三維結(jié)構(gòu),大小與原圖類似,其中通道數(shù)是類別數(shù),每個(gè)通道所標(biāo)記的像素點(diǎn),是該類別在圖像中的位置,最后通過argmax 取每個(gè)通道有用像素 合成一張圖像,用不同顏色表示其類別位置。 語義分割任務(wù)其實(shí)也是分類任務(wù)中的一種,他不過是對每一個(gè)像素點(diǎn)進(jìn)行細(xì)分,找到每一個(gè)像素點(diǎn)所述的類別。 這就是語義分割任務(wù)啦~ **
二、什么是deeplabv3
**DeepLabv3是一種語義分割架構(gòu),它在DeepLabv2的基礎(chǔ)上進(jìn)行了一些修改。為了處理在多個(gè)尺度上分割對象的問題,設(shè)計(jì)了在級聯(lián)或并行中采用多孔卷積的模塊,通過采用多個(gè)多孔速率來捕獲多尺度上下文。此外,來自 DeepLabv2 的 Atrous Spatial Pyramid Pooling模塊增加了編碼全局上下文的圖像級特征,并進(jìn)一步提高了性能。 **
三、LabVIEW調(diào)用DeepLabv3實(shí)現(xiàn)圖像語義分割
1、模型獲取及轉(zhuǎn)換
- 安裝pytorch和torchvision
- 獲取torchvision中的模型:deeplabv3_resnet101(我們獲取預(yù)訓(xùn)練好的模型):
original_model = models.segmentation.deeplabv3_resnet101(pretrained=True)
- 轉(zhuǎn)onnx
def get_pytorch_onnx_model(original_model):
# define the directory for further converted model save
onnx_model_path = dirname
# define the name of further converted model
onnx_model_name = "deeplabv3_resnet101.onnx"
?
# create directory for further converted model
os.makedirs(onnx_model_path, exist_ok=True)
?
# get full path to the converted model
full_model_path = os.path.join(onnx_model_path, onnx_model_name)
?
# generate model input
generated_input = Variable(
torch.randn(1, 3, 448, 448)
)
?
# model export into ONNX format
torch.onnx.export(
original_model,
generated_input,
full_model_path,
verbose=True,
input_names=["input"],
output_names=["output",'aux'],
opset_version=11
)
?
return full_model_path
完整獲取及模型轉(zhuǎn)換python代碼如下:
import os
import torch
import torch.onnx
from torch.autograd import Variable
from torchvision import models
import re
?
dirname, filename = os.path.split(os.path.abspath(__file__))
print(dirname)
?
def get_pytorch_onnx_model(original_model):
# define the directory for further converted model save
onnx_model_path = dirname
# define the name of further converted model
onnx_model_name = "deeplabv3_resnet101.onnx"
?
# create directory for further converted model
os.makedirs(onnx_model_path, exist_ok=True)
?
# get full path to the converted model
full_model_path = os.path.join(onnx_model_path, onnx_model_name)
?
# generate model input
generated_input = Variable(
torch.randn(1, 3, 448, 448)
)
?
# model export into ONNX format
torch.onnx.export(
original_model,
generated_input,
full_model_path,
verbose=True,
input_names=["input"],
output_names=["output",'aux'],
opset_version=11
)
?
return full_model_path
?
?
def main():
# initialize PyTorch ResNet-101 model
original_model = models.segmentation.deeplabv3_resnet101(pretrained=True)
?
# get the path to the converted into ONNX PyTorch model
full_model_path = get_pytorch_onnx_model(original_model)
print("PyTorch ResNet-101 model was successfully converted: ", full_model_path)
?
?
if __name__ == "__main__":
main()
?
我們會(huì)發(fā)現(xiàn),基于pytorch的DeepLabv3模型獲取和之前的mask rcnn模型大同小異。
2、關(guān)于deeplabv3_resnet101
我們使用的模型是:deeplabv3_resnet101,該模型返回兩個(gè)張量,與輸入張量相同,但有21個(gè)classes。輸出[“out”]包含語義掩碼,而輸出[“aux”]包含每像素的輔助損失值。在推理模式中,輸出[‘a(chǎn)ux]沒有用處。因此,輸出“out”形狀為(N、21、H、W)。我們在轉(zhuǎn)模型的時(shí)候設(shè)置H,W為448,N一般為1;
我們的模型是基于VOC2012數(shù)據(jù)集
VOC2012數(shù)據(jù)集分為20類,包括背景為21類,分別如下:
- 人 :人
- 動(dòng)物:鳥、貓、牛、狗、馬、羊
- 車輛:飛機(jī)、自行車、船、巴士、汽車、摩托車、火車
- 室內(nèi):瓶、椅子、餐桌、盆栽植物、沙發(fā)、電視/監(jiān)視器
3、LabVIEW opencv dnn調(diào)用 deeplabv3 實(shí)現(xiàn)圖像語義分割(deeplabv3_opencv.vi)
deeplabv3模型可以使用OpenCV dnn去加載的,也可以使用onnxruntime加載推理,所以我們分兩種方式給大家介紹LabVIEW調(diào)用deeplabv3實(shí)現(xiàn)圖像語義分割。
-
opencv dnn 調(diào)用onnx模型并選擇
-
**圖像預(yù)處理 **
最終還是采用了比較中規(guī)中矩的處理方式
- **執(zhí)行推理 **
-
后處理并實(shí)現(xiàn)實(shí)例分割
因?yàn)楹筇幚韮?nèi)容較多,所以直接封裝為了一個(gè)子VI, deeplabv3_postprocess.vi,因?yàn)長abview沒有專門的切片函數(shù),所以會(huì)稍慢一些,所以接下來還會(huì)開發(fā)針對后處理和矩陣有關(guān)的函數(shù),加快處理結(jié)果。
-
整體的程序框架如下:
-
語義分割結(jié)果如下:
4、LabVIEW onnxruntime調(diào)用 deeplabv3實(shí)現(xiàn)圖像語義分割 (deeplabv3_onnx.vi)
-
整體的程序框架如下:
-
語義分割結(jié)果如下:
5、LabVIEW onnxruntime調(diào)用 deeplabv3 使用TensorRT加速模型實(shí)現(xiàn)圖像語義分割(deeplabv3_onnx_camera.vi)
如上圖所示,可以看到可以把人和背景完全分割開來,使用TensorRT加速推理,速度也比較快。
大家可關(guān)注微信公眾號: VIRobotics ,回復(fù)關(guān)鍵字:DeepLabv3圖像語義分割源碼 獲取本次分享內(nèi)容的完整項(xiàng)目源碼及模型。
如您想要探討更多關(guān)于LabVIEW與人工智能技術(shù),歡迎加入我們:705637299,進(jìn)群請備注暗號:LabVIEW機(jī)器學(xué)習(xí)
四、deeplabv3訓(xùn)練自己的數(shù)據(jù)集
訓(xùn)練可參考: https://github.com/pytorch/vision
總結(jié)
以上就是今天要給大家分享的內(nèi)容。
如果文章對你有幫助,歡迎關(guān)注、點(diǎn)贊、收藏
審核編輯 黃宇
-
LabVIEW
+關(guān)注
關(guān)注
1975文章
3656瀏覽量
324638 -
人工智能
+關(guān)注
關(guān)注
1792文章
47514瀏覽量
239247 -
機(jī)器學(xué)習(xí)
+關(guān)注
關(guān)注
66文章
8428瀏覽量
132845 -
深度學(xué)習(xí)
+關(guān)注
關(guān)注
73文章
5510瀏覽量
121347 -
pytorch
+關(guān)注
關(guān)注
2文章
808瀏覽量
13290
發(fā)布評論請先 登錄
相關(guān)推薦
van-自然和醫(yī)學(xué)圖像的深度語義分割:網(wǎng)絡(luò)結(jié)構(gòu)
van-自然和醫(yī)學(xué)圖像的深度語義分割:網(wǎng)絡(luò)結(jié)構(gòu)
聚焦語義分割任務(wù),如何用卷積神經(jīng)網(wǎng)絡(luò)處理語義圖像分割?
DeepLab進(jìn)行語義分割的研究分析
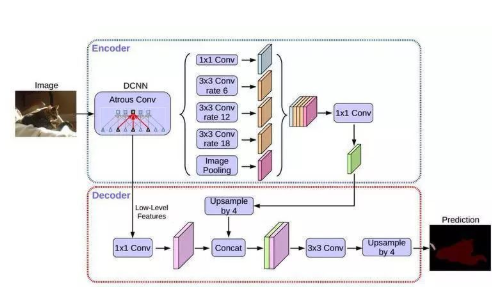
分析總結(jié)基于深度神經(jīng)網(wǎng)絡(luò)的圖像語義分割方法
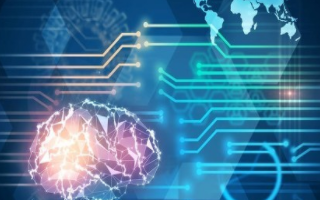
基于深度神經(jīng)網(wǎng)絡(luò)的圖像語義分割方法
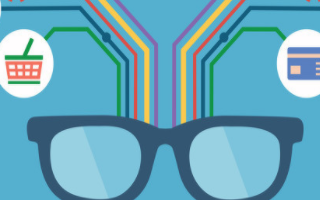
輕松學(xué)Pytorch之Deeplabv3推理
PyTorch教程14.9之語義分割和數(shù)據(jù)集
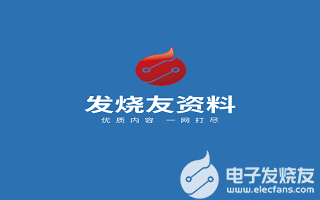
PyTorch教程-14.9. 語義分割和數(shù)據(jù)集
DeepLabV3開發(fā)板應(yīng)用
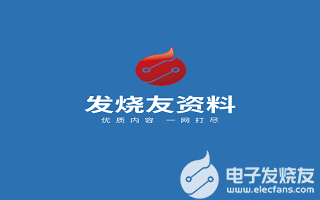
評論