實(shí)現(xiàn)原理
與DataParallel不同的是,Distributed Data Parallel會(huì)開(kāi)設(shè)多個(gè)進(jìn)程而非線程,進(jìn)程數(shù) = GPU數(shù),每個(gè)進(jìn)程都可以獨(dú)立進(jìn)行訓(xùn)練,也就是說(shuō)代碼的所有部分都會(huì)被每個(gè)進(jìn)程同步調(diào)用,如果你某個(gè)地方print張量,你會(huì)發(fā)現(xiàn)device的差異
sampler會(huì)將數(shù)據(jù)按照進(jìn)程數(shù)切分,
「確保不同進(jìn)程的數(shù)據(jù)不同」
每個(gè)進(jìn)程獨(dú)立進(jìn)行前向訓(xùn)練
每個(gè)進(jìn)程利用Ring All-Reduce進(jìn)行通信,將梯度信息進(jìn)行聚合
每個(gè)進(jìn)程同步更新模型參數(shù),進(jìn)行新一輪訓(xùn)練
按進(jìn)程切分
如何確保數(shù)據(jù)不同呢?不妨看看DistributedSampler的源碼
#判斷數(shù)據(jù)集長(zhǎng)度是否可以整除GPU數(shù) #如果不能,選擇舍棄還是補(bǔ)全,進(jìn)而決定總數(shù) #Ifthedatasetlengthisevenlydivisibleby#ofreplicas #thenthereisnoneedtodropanydata,sincethedataset #willbesplitequally. if(self.drop_lastand len(self.dataset)%self.num_replicas!=0): #num_replicas=num_gpus self.num_samples=math.ceil((len(self.dataset)- self.num_replicas)/self.num_replicas) else: self.num_samples=math.ceil(len(self.dataset)/ self.num_replicas) self.total_size=self.num_samples*self.num_replicas #根據(jù)是否shuffle來(lái)創(chuàng)建indices ifself.shuffle: #deterministicallyshufflebasedonepochandseed g=torch.Generator() g.manual_seed(self.seed+self.epoch) indices=torch.randperm(len(self.dataset),generator=g).tolist() else: indices=list(range(len(self.dataset))) ifnotself.drop_last: #addextrasamplestomakeitevenlydivisible padding_size=self.total_size-len(indices) ifpadding_size<=?len(indices): ????????#?不夠就按indices順序加 ????????#?e.g.,?indices為[0,?1,?2,?3?...],而padding_size為4 ????????#?加好之后的indices[...,?0,?1,?2,?3] ????????indices?+=?indices[:padding_size] ????else: ????????indices?+=?(indices?*?math.ceil(padding_size?/?len(indices)))[:padding_size] else: ????#?remove?tail?of?data?to?make?it?evenly?divisible. ????indices?=?indices[:self.total_size] assert?len(indices)?==?self.total_size #?subsample #?rank代表進(jìn)程id indices?=?indices[self.rankself.num_replicas] return?iter(indices)
Ring All-Reduce
那么什么是「Ring All-Reduce」呢?又為啥可以降低通信成本呢?
首先將每塊GPU上的梯度拆分成四個(gè)部分,比如,如下圖(此部分原理致謝下王老師,講的很清晰[1]:
所有GPU的傳播都是「同步」進(jìn)行的,傳播的規(guī)律有兩條:
只與自己下一個(gè)位置的GPU進(jìn)行通信,比如0 > 1,3 > 0
四個(gè)部分,哪塊GPU上占的多,就由該塊GPU往它下一個(gè)傳,初始從主節(jié)點(diǎn)傳播,即GPU0,你可以想象跟接力一樣,a傳b,b負(fù)責(zé)傳給c
第一次傳播如下:
那么結(jié)果就是:
那么,按照誰(shuí)多誰(shuí)往下傳的原則,此時(shí)應(yīng)該是GPU1往GPU2傳a0和a1,GPU2往GPU3傳b1和b2,以此類推
接下來(lái)再傳播就會(huì)有GPU3 a的部分全有,GPU0上b的部分全有等,就再往下傳
再來(lái)幾遍便可以使得每塊GPU上都獲得了來(lái)自其他GPU的梯度啦
代碼使用
基礎(chǔ)概念
第一個(gè)是后端的選擇,即數(shù)據(jù)傳輸協(xié)議,從下表可以看出[2],當(dāng)使用CPU時(shí)可以選擇gloo而GPU則可以是nccl
「Backend」 | 「gloo」 | 「mpi」 | 「nccl」 | |||
---|---|---|---|---|---|---|
Device | CPU | GPU | CPU | GPU | CPU | GPU |
send | ? | ? | ? | ? | ? | ? |
recv | ? | ? | ? | ? | ? | ? |
broadcast | ? | ? | ? | ? | ? | ? |
all_reduce | ? | ? | ? | ? | ? | ? |
reduce | ? | ? | ? | ? | ? | ? |
all_gather | ? | ? | ? | ? | ? | ? |
gather | ? | ? | ? | ? | ? | ? |
scatter | ? | ? | ? | ? | ? | ? |
reduce_scatter | ? | ? | ? | ? | ? | ? |
all_to_all | ? | ? | ? | ? | ? | ? |
barrier | ? | ? | ? | ? | ? | ? |
接下來(lái)是一些參數(shù)的解釋[3]:
Arg | Meaning |
---|---|
group | 一次發(fā)起的所有進(jìn)程構(gòu)成一個(gè)group,除非想更精細(xì)通信,創(chuàng)建new_group |
world_size | 一個(gè)group中進(jìn)程數(shù)目,即為GPU的數(shù)量 |
rank | 進(jìn)程id,主節(jié)點(diǎn)rank=0,其他的在0和world_size-1之間 |
local_rank | 進(jìn)程在本地節(jié)點(diǎn)/機(jī)器的id |
舉個(gè)例子,假如你有兩臺(tái)服務(wù)器(又被稱為node),每臺(tái)服務(wù)器有4張GPU,那么,world_size即為8,rank=[0, 1, 2, 3, 4, 5, 6, 7], 每個(gè)服務(wù)器上的進(jìn)程的local_rank為[0, 1, 2, 3]
然后是「初始化方法」的選擇,有TCP和共享文件兩種,一般指定rank=0為master節(jié)點(diǎn)
TCP顯而易見(jiàn)是通過(guò)網(wǎng)絡(luò)進(jìn)行傳輸,需要指定主節(jié)點(diǎn)的ip(可以為主節(jié)點(diǎn)實(shí)際IP,或者是localhost)和空閑的端口
importtorch.distributedasdist dist.init_process_group(backend,init_method='tcp://ip:port', rank=rank,world_size=world_size)
共享文件的話需要手動(dòng)刪除上次啟動(dòng)時(shí)殘留的文件,加上官方有一堆警告,還是建議使用TCP
dist.init_process_group(backend,init_method='file://Path', rank=rank,world_size=world_size)
launch方法
「初始化」
這里先講用launch的方法,關(guān)于torch.multiprocessing留到后面講
在啟動(dòng)后,rank和world_size都會(huì)自動(dòng)被DDP寫入環(huán)境中,可以提前準(zhǔn)備好參數(shù)類,如argparse這種
args.rank=int(os.environ['RANK']) args.world_size=int(os.environ['WORLD_SIZE']) args.local_rank=int(os.environ['LOCAL_RANK'])
首先,在使用distributed包的任何其他函數(shù)之前,按照tcp方法進(jìn)行初始化,需要注意的是需要手動(dòng)指定一共可用的設(shè)備CUDA_VISIBLE_DEVICES
defdist_setup_launch(args): #tellDDPavailabledevices[NECESSARY] os.environ['CUDA_VISIBLE_DEVICES']=args.devices args.rank=int(os.environ['RANK']) args.world_size=int(os.environ['WORLD_SIZE']) args.local_rank=int(os.environ['LOCAL_RANK']) dist.init_process_group(args.backend, args.init_method, rank=args.rank, world_size=args.world_size) #thisisoptional,otherwiseyoumayneedtospecifythe #devicewhenyoumovesomethinge.g.,model.cuda(1) #ormodel.to(args.rank) #Settingdevicemakesthingseasy:model.cuda() torch.cuda.set_device(args.rank) print('TheCurrentRankis%d|TheTotalRanksare%d' %(args.rank,args.world_size))
「DistributedSampler」
接下來(lái)創(chuàng)建DistributedSampler,是否pin_memory,根據(jù)你本機(jī)的內(nèi)存決定。pin_memory的意思是提前在內(nèi)存中申請(qǐng)一部分專門存放Tensor。假如說(shuō)你內(nèi)存比較小,就會(huì)跟虛擬內(nèi)存,即硬盤進(jìn)行交換,這樣轉(zhuǎn)義到GPU上會(huì)比內(nèi)存直接到GPU耗時(shí)。
因而,如果你的內(nèi)存比較大,可以設(shè)置為True;然而,如果開(kāi)了導(dǎo)致卡頓的情況,建議關(guān)閉
fromtorch.utils.dataimportDataLoader,DistributedSampler train_sampler=DistributedSampler(train_dataset,seed=args.seed) train_dataloader=DataLoader(train_dataset, pin_memory=True, shuffle=(train_samplerisNone), batch_size=args.per_gpu_train_bs, num_workers=args.num_workers, sampler=train_sampler) eval_sampler=DistributedSampler(eval_dataset,seed=args.seed) eval_dataloader=DataLoader(eval_dataset, pin_memory=True, batch_size=args.per_gpu_eval_bs, num_workers=args.num_workers, sampler=eval_sampler)
「加載模型」
然后加載模型,跟DataParallel不同的是需要提前放置到cuda上,還記得上面關(guān)于設(shè)置cuda_device的語(yǔ)句嘛,因?yàn)樵O(shè)置好之后每個(gè)進(jìn)程只能看見(jiàn)一個(gè)GPU,所以直接model.cuda(),不需要指定device
同時(shí),我們必須給DDP提示目前是哪個(gè)rank
fromtorch.nn.parallelimportDistributedDataParallelasDDP model=model.cuda() #tellDDPwhichrank model=DDP(model,find_unused_parameters=True,device_ids=[rank])
注意,當(dāng)模型帶有Batch Norm時(shí):
ifargs.syncBN: nn.SyncBatchNorm.convert_sync_batchnorm(model).cuda()
「訓(xùn)練相關(guān)」
每個(gè)epoch開(kāi)始訓(xùn)練的時(shí)候,記得用sampler的set_epoch,這樣使得每個(gè)epoch打亂順序是不一致的
關(guān)于梯度回傳和參數(shù)更新,跟正常情況無(wú)異
forepochinrange(epochs): #recordepochs train_dataloader.sampler.set_epoch(epoch) outputs=model(inputs) loss=loss_fct(outputs,labels) loss.backward() optimizer.step() optimizer.zero_grad()
這里有一點(diǎn)需要小心,這個(gè)loss是各個(gè)進(jìn)程的loss之和,如果想要存儲(chǔ)每個(gè)step平均損失,可以進(jìn)行all_reduce操作,進(jìn)行平均,不妨看官方的小例子來(lái)理解下:
>>>#Alltensorsbelowareoftorch.int64type. >>>#Wehave2processgroups,2ranks. >>>tensor=torch.arange(2,dtype=torch.int64)+1+2*rank >>>tensor tensor([1,2])#Rank0 tensor([3,4])#Rank1 >>>dist.all_reduce(tensor,op=ReduceOp.SUM) >>>tensor tensor([4,6])#Rank0 tensor([4,6])#Rank1
@torch.no_grad() defreduce_value(value,average=True): world_size=get_world_size() ifworld_size2:??#?單GPU的情況 ????????return?value ????dist.all_reduce(value) ????if?average: ?????value?/=?world_size ????return?value
看到這,肯定有小伙伴要問(wèn),那這樣我們是不是得先求平均損失再回傳梯度啊,不用,因?yàn)?,?dāng)我們回傳loss后,DDP會(huì)自動(dòng)對(duì)所有梯度進(jìn)行平均[4],也就是說(shuō)回傳后我們更新的梯度和DP或者單卡同樣batch訓(xùn)練都是一致的
loss=loss_fct(...) loss.backward() #注意在backward后面 loss=reduce_value(loss,world_size) mean_loss=(step*mean_loss+loss.item())/(step+1)
還有個(gè)注意點(diǎn)就是學(xué)習(xí)率的變化,這個(gè)是和batch size息息相關(guān)的,如果batch擴(kuò)充了幾倍,也就是說(shuō)step比之前少了很多,還采用同一個(gè)學(xué)習(xí)率,肯定會(huì)出問(wèn)題的,這里,我們進(jìn)行線性增大[5]
N=world_size lr=args.lr*N
肯定有人說(shuō),誒,你線性增大肯定不能保證梯度的variance一致了,正確的應(yīng)該是正比于,關(guān)于這個(gè)的討論不妨參考[6]
「evaluate相關(guān)」
接下來(lái),細(xì)心的同學(xué)肯定好奇了,如果驗(yàn)證集也切分了,metric怎么計(jì)算呢?此時(shí)就需要咱們把每個(gè)進(jìn)程得到的預(yù)測(cè)情況集合起來(lái),t就是一個(gè)我們需要gather的張量,最后將每個(gè)進(jìn)程中的t按照第一維度拼接,先看官方小例子來(lái)理解all_gather
>>>#Alltensorsbelowareoftorch.int64dtype. >>>#Wehave2processgroups,2ranks. >>>tensor_list=[torch.zeros(2,dtype=torch.int64)for_inrange(2)] >>>tensor_list [tensor([0,0]),tensor([0,0])]#Rank0and1 >>>tensor=torch.arange(2,dtype=torch.int64)+1+2*rank >>>tensor tensor([1,2])#Rank0 tensor([3,4])#Rank1 >>>dist.all_gather(tensor_list,tensor) >>>tensor_list [tensor([1,2]),tensor([3,4])]#Rank0 [tensor([1,2]),tensor([3,4])]#Rank1
defsync_across_gpus(t,world_size): gather_t_tensor=[torch.zeros_like(t)for_in range(world_size)] dist.all_gather(gather_t_tensor,t) returntorch.cat(gather_t_tensor,dim=0)
可以簡(jiǎn)單參考我前面提供的源碼的evaluate部分,我們首先將預(yù)測(cè)和標(biāo)簽比對(duì),把結(jié)果為bool的張量存儲(chǔ)下來(lái),最終gather求和取平均。
這里還有個(gè)有趣的地方,tensor默認(rèn)的類型可能是int,bool型的res拼接后自動(dòng)轉(zhuǎn)為0和1了,另外bool型的張量是不支持gather的
defeval(...) results=torch.tensor([]).cuda() forstep,(inputs,labels)inenumerate(dataloader): outputs=model(inputs) res=(outputs.argmax(-1)==labels) results=torch.cat([results,res],dim=0) results=sync_across_gpus(results,world_size) mean_acc=(results.sum()/len(results)).item() returnmean_acc
「模型保存與加載」
模型保存,參考部分官方教程[7],我們只需要在主進(jìn)程保存模型即可,注意,這里是被DDP包裹后的,DDP并沒(méi)有state_dict,這里barrier的目的是為了讓其他進(jìn)程等待主進(jìn)程保存模型,以防不同步
defsave_checkpoint(rank,model,path): ifis_main_process(rank): #Allprocessesshouldseesameparametersastheyall #startfromsamerandomparametersandgradientsare #synchronizedinbackwardpasses. #Therefore,savingitinoneprocessissufficient. torch.save(model.module.state_dict(),path) #Useabarrier()tokeepprocess1waitingforprocess0 dist.barrier()
加載的時(shí)候別忘了map_location,我們一開(kāi)始會(huì)保存模型至主進(jìn)程,這樣就會(huì)導(dǎo)致cuda:0顯存被占據(jù),我們需要將模型remap到其他設(shè)備
defload_checkpoint(rank,model,path): #remapthemodelfromcuda:0tootherdevices map_location={'cuda:%d'%0:'cuda:%d'%rank} model.module.load_state_dict( torch.load(path,map_location=map_location) )
進(jìn)程銷毀
運(yùn)行結(jié)束后記得銷毀進(jìn)程:
defcleanup(): dist.destroy_process_group() cleanup()
如何啟動(dòng)
在終端輸入下列命令【單機(jī)多卡】
python-mtorch.distributed.launch--nproc_per_node=NUM_GPUS main.py(--arg1--arg2--arg3andallother argumentsofyourtrainingscript)
目前torch 1.10以后更推薦用run
torch.distributed.launch->torch.distributed.run/torchrun
多機(jī)多卡是這樣的:
#第一個(gè)節(jié)點(diǎn)啟動(dòng) python-mtorch.distributed.launch --nproc_per_node=NUM_GPUS --nnodes=2 --node_rank=0 --master_addr="192.168.1.1" --master_port=1234main.py #第二個(gè)節(jié)點(diǎn)啟動(dòng) python-mtorch.distributed.launch --nproc_per_node=NUM_GPUS --nnodes=2 --node_rank=1 --master_addr="192.168.1.1" --master_port=1234main.py
mp方法
第二個(gè)方法就是利用torch的多線程包
importtorch.multiprocessingasmp #rankmp會(huì)自動(dòng)填入 defmain(rank,arg1,...): pass if__name__=='__main__': mp.spawn(main,nprocs=TOTAL_GPUS,args=(arg1,...))
這種運(yùn)行的時(shí)候就跟正常的python文件一致:
pythonmain.py
優(yōu)缺點(diǎn)
「優(yōu)點(diǎn)」:相比于DP而言,不需要反復(fù)創(chuàng)建和銷毀線程;Ring-AllReduce算法提高通信效率;模型同步方便
「缺點(diǎn)」:操作起來(lái)可能有些復(fù)雜,一般可滿足需求的可先試試看DataParallel。
審核編輯:劉清
-
gpu
+關(guān)注
關(guān)注
28文章
4766瀏覽量
129194 -
PIN管
+關(guān)注
關(guān)注
0文章
36瀏覽量
6366 -
TCP通信
+關(guān)注
關(guān)注
0文章
146瀏覽量
4270
原文標(biāo)題:深入理解Pytorch中的分布式訓(xùn)練
文章出處:【微信號(hào):zenRRan,微信公眾號(hào):深度學(xué)習(xí)自然語(yǔ)言處理】歡迎添加關(guān)注!文章轉(zhuǎn)載請(qǐng)注明出處。
發(fā)布評(píng)論請(qǐng)先 登錄
相關(guān)推薦
分布式云化數(shù)據(jù)庫(kù)有哪些類型
基于ptp的分布式系統(tǒng)設(shè)計(jì)
HarmonyOS Next 應(yīng)用元服務(wù)開(kāi)發(fā)-分布式數(shù)據(jù)對(duì)象遷移數(shù)據(jù)文件資產(chǎn)遷移
HarmonyOS Next 應(yīng)用元服務(wù)開(kāi)發(fā)-分布式數(shù)據(jù)對(duì)象遷移數(shù)據(jù)權(quán)限與基礎(chǔ)數(shù)據(jù)
分布式通信的原理和實(shí)現(xiàn)高效分布式通信背后的技術(shù)NVLink的演進(jìn)
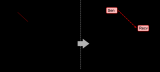
分布式光纖聲波傳感技術(shù)的工作原理
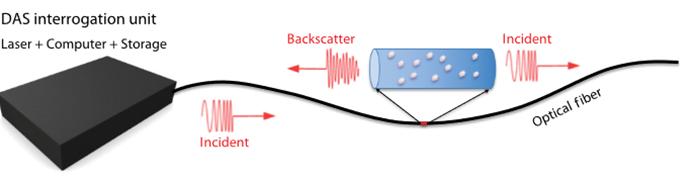
分布式輸電線路故障定位中的分布式是指什么
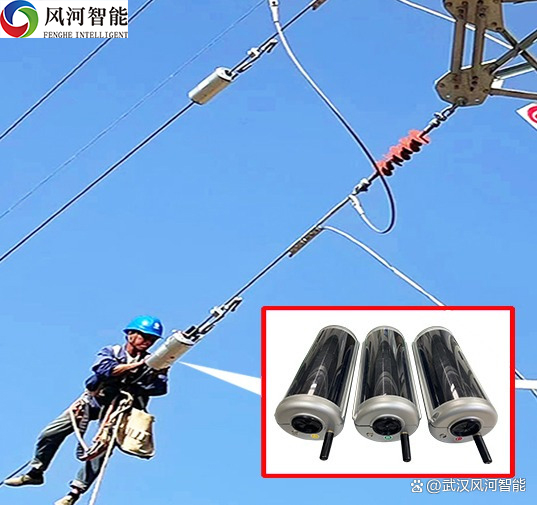
分布式SCADA系統(tǒng)的特點(diǎn)的組成
鴻蒙開(kāi)發(fā)接口數(shù)據(jù)管理:【@ohos.data.distributedData (分布式數(shù)據(jù)管理)】
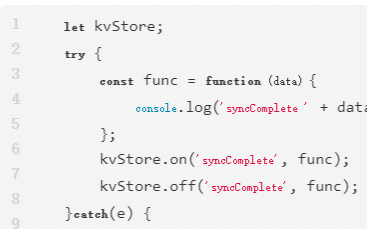
HarmonyOS開(kāi)發(fā)實(shí)例:【分布式數(shù)據(jù)服務(wù)】
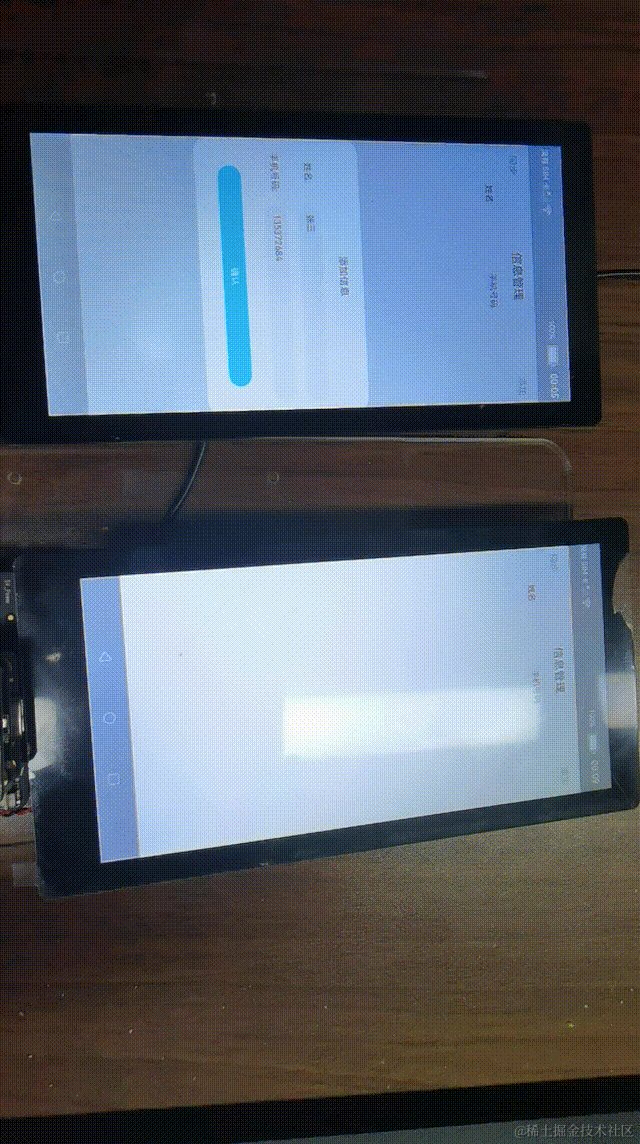
HarmonyOS實(shí)戰(zhàn)案例:【分布式賬本】
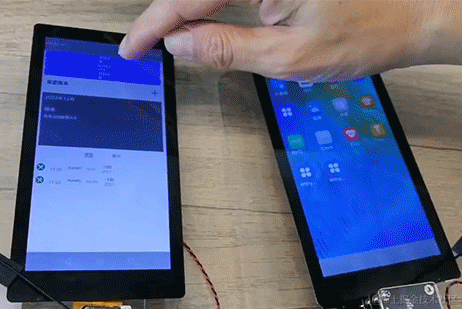
評(píng)論