在 xgboost1.0 中,我們引入了 新的官方 Dask 接口 來支持高效的分布式訓(xùn)練。 快速轉(zhuǎn)發(fā)到 XGBoost1.4 ,接口現(xiàn)在功能齊全。如果您對 xgboostdask 接口還不熟悉,請參閱第一篇文章,以獲得一個溫和的介紹。在本文中,我們將看一些簡單的代碼示例,展示如何最大化GPU加速的好處。
我們的例子集中在希格斯數(shù)據(jù)集上,這是一個來自 機(jī)器學(xué)習(xí)庫 的中等規(guī)模的分類問題。 在下面的章節(jié)中,我們從基本數(shù)據(jù)加載和預(yù)處理開始,使用 GPU 加速的 Dask 和 Dask-ml 。然后,針對不同配置的返回數(shù)據(jù)訓(xùn)練 XGBoost 模型。同時,分享一些新特性。之后,我們將展示如何在 GPU 集群上計算 SHAP 值以及可以獲得的加速比。最后,我們分享了一些優(yōu)化技術(shù)與推理。
以下示例需要在至少有一個 NVIDIA GPU 的機(jī)器上運行, GPU 可以是筆記本電腦或云實例。 Dask 的優(yōu)點之一是它的靈活性,用戶可以在筆記本電腦上測試他們的代碼。它們還可以將計算擴(kuò)展到具有最小代碼更改量的集群。 另外,要設(shè)置環(huán)境,我們需要 xgboost==1.4 、 dask 、 dask-ml 、 dask-cuda 和 達(dá)斯克 – cuDF python 包,可從 RAPIDS 康達(dá)頻道: 獲得
conda install -c rapidsai -c conda-forge dask dask-ml dask-cuda dask-cudf xgboost=1.4.2
在 GPU 集群上用 Dask 加載數(shù)據(jù)
首先,我們將數(shù)據(jù)集下載到 data 目錄中。
mkdir data
curl http://archive.ics.uci.edu/ml/machine-learning-databases/00280/HIGGS.csv.gz --output ./data/HIGGS.csv.gz
然后使用dask-cuda設(shè)置 GPU 集群:
import os
from time import time
from typing import Tuple
from dask import dataframe as dd
from dask_cuda import LocalCUDACluster
from distributed import Client, wait
import dask_cudf
from dask_ml.model_selection import train_test_split
import xgboost as xgb
from xgboost import dask as dxgb
import numpy as np
import argparse
# … main content to be inserted here in the following sections
if __name__ == "__main__":
parser = argparse.ArgumentParser()
parser.add_argument("--n_workers", type=int, required=True)
args = parser.parse_args()
with LocalCUDACluster(args.n_workers) as cluster:
print("dashboard:", cluster.dashboard_link)
with Client(cluster) as client:
main(client)
給定一個集群,我們開始將數(shù)據(jù)加載到 gpu 中。 由于在參數(shù)調(diào)整期間多次加載數(shù)據(jù),因此我們將 CSV 文件轉(zhuǎn)換為 Parquet 格式以獲得更好的性能。 這可以使用dask_cudf輕松完成:
def to_parquet() -> str:
"""Convert the HIGGS.csv file to parquet files."""
dirpath = "./data"
parquet_path = os.path.join(dirpath, "HIGGS.parquet")
if os.path.exists(parquet_path):
return parquet_path
csv_path = os.path.join(dirpath, "HIGGS.csv")
colnames = ["label"] + ["feature-%02d" % i for i in range(1, 29)]
df = dask_cudf.read_csv(csv_path, header=None, names=colnames, dtype=np.float32)
df.to_parquet(parquet_path)
return parquet_path
數(shù)據(jù)加載后,我們準(zhǔn)備培訓(xùn)/驗證拆分:
def load_higgs(
path,
) -> Tuple[
dask_cudf.DataFrame, dask_cudf.Series, dask_cudf.DataFrame, dask_cudf.Series
]:
df = dask_cudf.read_parquet(path)
y = df["label"]
X = df[df.columns.difference(["label"])]
X_train, X_valid, y_train, y_valid = train_test_split(
X, y, test_size=0.33, random_state=42
)
X_train, X_valid, y_train, y_valid = client.persist(
[X_train, X_valid, y_train, y_valid]
)
wait([X_train, X_valid, y_train, y_valid])
return X_train, X_valid, y_train, y_valid
在前面的示例中,我們使用 dask-cudf 從磁盤加載數(shù)據(jù),使用 dask-ml 中的 火車測試分裂了 函數(shù)拆分?jǐn)?shù)據(jù)集。 大多數(shù)時候, dask 的 GPU 后端與 dask-ml 中的實用程序無縫地工作,我們可以加速整個 ML 管道。
提前停止訓(xùn)練
最常請求的特性之一是提前停止對 Dask 接口的支持。 在 XGBoost1 。 4 版本中,我們不僅可以指定停止輪的數(shù)量,還可以開發(fā)定制的提前停止策略。 對于最簡單的情況,向 train 函數(shù)提供停止回合可以實現(xiàn)提前停止:
def fit_model_es(client, X, y, X_valid, y_valid) -> xgb.Booster:
early_stopping_rounds = 5
Xy = dxgb.DaskDeviceQuantileDMatrix(client, X, y)
Xy_valid = dxgb.DaskDMatrix(client, X_valid, y_valid)
# train the model
booster = dxgb.train(
client,
{
"objective": "binary:logistic",
"eval_metric": "error",
"tree_method": "gpu_hist",
},
Xy,
evals=[(Xy_valid, "Valid")],
num_boost_round=1000,
early_stopping_rounds=early_stopping_rounds,
)["booster"]
return booster
在前面的片段中有兩件事需要注意。 首先,我們指定觸發(fā)提前停止訓(xùn)練的輪數(shù)。 XGBoost 將在連續(xù) X 輪驗證指標(biāo)未能改善時停止培訓(xùn)過程,其中 X 是指定提前停止的輪數(shù)。 其次,我們使用名為 DaskDeviceQuantileDMatrix 的數(shù)據(jù)類型進(jìn)行訓(xùn)練,但使用 DaskDMatrix 進(jìn)行驗證。 DaskDeviceQuantileDMatrix 是 DaskDMatrix 的替代品,用于基于 GPU 的訓(xùn)練輸入,避免了額外的數(shù)據(jù)拷貝。
與 gpu_hist 一起使用時, DaskDeviceQuantileDMatrix 可以節(jié)省大量內(nèi)存,并且輸入數(shù)據(jù)已經(jīng)在 GPU 上。圖 1 描述了 DaskDeviceQuantileDMatrix. 的結(jié)構(gòu) 數(shù)據(jù)分區(qū)不再需要復(fù)制和連接,取而代之的是,由草圖算法生成的摘要被用作真實數(shù)據(jù)的代理。
圖 1 : DaskDeviceQuantileDMatrix 的構(gòu)造 。
在 XGBoost 中,提前停止作為回調(diào)函數(shù)實現(xiàn)。 新的回調(diào)接口可以用來實現(xiàn)更高級的提前停止策略。下面的代碼顯示了提前停止的另一種實現(xiàn),其中有一個附加參數(shù)要求 XGBoost 僅返回最佳模型,而不是完整模型:
def fit_model_customized_es(client, X, y, X_valid, y_valid): early_stopping_rounds = 5 es = xgb.callback.EarlyStopping(rounds=early_stopping_rounds, save_best=True) Xy = dxgb.DaskDeviceQuantileDMatrix(client, X, y) Xy_valid = dxgb.DaskDMatrix(client, X_valid, y_valid) # train the model booster = xgb.dask.train( client, { "objective": "binary:logistic", "eval_metric": "error", "tree_method": "gpu_hist", }, Xy, evals=[(Xy_valid, "Valid")], num_boost_round=1000, callbacks=[es], )["booster"] return booster
在前面的示例中, EarlyStopping 回調(diào)作為參數(shù)提供給 train ,而不是使用 early_stopping_rounds 參數(shù)。為了提供一個定制的提前停止策略,探索 EarlyStopping 的其他參數(shù)或子類化這個回調(diào)是一個很好的起點。
定制目標(biāo)和評估指標(biāo)
XGBoost 被設(shè)計成可以通過定制的目標(biāo)函數(shù)和度量進(jìn)行擴(kuò)展。在 1 。 4 中,這個特性被引入 dask 接口。要求與單節(jié)點接口完全相同:
def fit_model_customized_objective(client, X, y, X_valid, y_valid) -> dxgb.Booster:
def logit(predt: np.ndarray, Xy: xgb.DMatrix) -> Tuple[np.ndarray, np.ndarray]:
predt = 1.0 / (1.0 + np.exp(-predt))
labels = Xy.get_label()
grad = predt - labels
hess = predt * (1.0 - predt)
return grad, hess
def error(predt: np.ndarray, Xy: xgb.DMatrix) -> Tuple[str, float]:
label = Xy.get_label()
r = np.zeros(predt.shape)
predt = 1.0 / (1.0 + np.exp(-predt))
gt = predt > 0.5
r[gt] = 1 - label[gt]
le = predt <= 0.5
r[le] = label[le]
return "CustomErr", float(np.average(r))
# Use early stopping with custom objective and metric.
early_stopping_rounds = 5
# Specify the metric we want to use for early stopping.
es = xgb.callback.EarlyStopping(
rounds=early_stopping_rounds, save_best=True, metric_name="CustomErr"
)
Xy = dxgb.DaskDeviceQuantileDMatrix(client, X, y)
Xy_valid = dxgb.DaskDMatrix(client, X_valid, y_valid)
booster = dxgb.train(
client,
{"eval_metric": "error", "tree_method": "gpu_hist"},
Xy,
evals=[(Xy_valid, "Valid")],
num_boost_round=1000,
obj=logit, # pass the custom objective
feval=error, # pass the custom metric
callbacks=[es],
)["booster"]
return booster
在前面的函數(shù)中,我們使用定制的目標(biāo)函數(shù)和度量來實現(xiàn)一個 logistic 回歸模型以及提前停止。請注意,該函數(shù)同時返回 gradient 和 hessian , XGBoost 使用它們來優(yōu)化模型。 另外,需要在回調(diào)中指定名為 metric_name 的參數(shù)。它用于通知 XGBoost 應(yīng)該使用自定義錯誤函數(shù)來評估早期停止標(biāo)準(zhǔn)。
解釋模型
在得到我們的第一個模型之后,我們 MIG ht 想用 SHAP 來解釋預(yù)測。 SHapley 加法解釋( SHapley Additive explainstructions , SHapley Additive explainstructions )是一種基于 SHapley 值解釋機(jī)器學(xué)習(xí)模型輸出的博弈論方法。 有關(guān)算法的詳細(xì)信息,請參閱 papers 。 由于 XGBoost 現(xiàn)在支持 GPU 加速的 Shapley 值,因此我們將此功能擴(kuò)展到 Dask 接口。現(xiàn)在,用戶可以在分布式 GPU 集群上計算 shap 值。這是由顯著改進(jìn)的預(yù)測函數(shù)和 GPUTreeShap 庫 實現(xiàn)的:
def explain(client, model, X):
# Use array instead of dataframe in case of output dim is greater than 2.
X_array = X.values
contribs = dxgb.predict(
client, model, X_array, pred_contribs=True, validate_features=False
)
# Use the result for further analysis
return contribs
XGBoost 使用多個 GPU 計算 shap 值的性能如圖 2 所示。
圖 2 : Shap 推斷時間。
基準(zhǔn)測試是在一臺 NVIDIA DGX-1 服務(wù)器上進(jìn)行的,該服務(wù)器有 8 個 V100 gpu 和兩個 20 核的 Xeon E5-2698 v4 cpu ,并進(jìn)行了一輪訓(xùn)練、 shap 值計算和推理。
得到的 SHAP 值可用于可視化、使用特征權(quán)重調(diào)整列采樣或用于其他數(shù)據(jù)工程目的。
運行推理
經(jīng)過一些調(diào)整,我們得到了對新數(shù)據(jù)進(jìn)行推理的最終模型。 XGBoost Dask 接口的預(yù)測沒有舊版本那么有效,而且內(nèi)存不足。在 1 。 4 中,我們修改了預(yù)測函數(shù)并增加了對就地預(yù)測的支持。 對于正態(tài)預(yù)測,它使用與 shap 值計算相同的接口:
def predict(client, model, X):
predt = dxgb.predict(client, model, X)
assert isinstance(predt, dd.Series)
return predt
標(biāo)準(zhǔn)的 predict 函數(shù)提供了一個通用接口,可同時接受DaskDMatrix和 dask 集合(數(shù)據(jù)幀或數(shù)組),但沒有針對內(nèi)存使用進(jìn)行優(yōu)化。在這里,我們將其替換為就地預(yù)測,它支持基本的推理任務(wù),并且不需要將數(shù)據(jù)復(fù)制到 XGBoost 的內(nèi)部數(shù)據(jù)結(jié)構(gòu)中:
def inplace_predict(client, model, X):
# Use inplace_predict instead of standard predict.
predt = dxgb.inplace_predict(client, model, X)
assert isinstance(predt, dd.Series)
return predt
內(nèi)存節(jié)省取決于每個塊的大小和輸入類型。當(dāng)使用同一模型多次運行推理時,另一個潛在的優(yōu)化是對模型進(jìn)行預(yù)格式化。默認(rèn)情況下,每次調(diào)用predict時, XGBoost 都會將模型傳輸給 worker ,從而產(chǎn)生大量開銷。好消息是 Dask 函數(shù)接受 future 對象作為完成模型的代理。然后我們可以傳輸數(shù)據(jù),這些數(shù)據(jù)可以與其他計算和持久化數(shù)據(jù)重疊。
def inplace_predict_multi_parts(client, model, X_train, X_valid):
"""Simulate the scenario that we need to run prediction on multiple datasets using train
and valid. In real world the number of datasets is unlimited
"""
# prescatter the model onto workers
model_f = client.scatter(model)
predictions = []
for X in [X_train, X_valid]:
# Use inplace_predict instead of standard predict.
predt = dxgb.inplace_predict(client, model_f, X)
assert isinstance(predt, dd.Series)
predictions.append(predt)
return predictions
在前面的代碼片段中,我們將未來的模型傳遞給 XGBoost ,而不是真正的模型。 這樣我們就避免了在預(yù)測過程中的重復(fù)傳輸,或者我們可以將模型傳輸與其他操作(如加載數(shù)據(jù))并行,如注釋中所建議的那樣。
把它們放在一起
在前面的部分中,我們將演示早期停止、形狀值計算、自定義目標(biāo)以及最終推斷。下表顯示了具有不同工作線程數(shù)的 GPU 集群的端到端加速。
圖 3 : GPU 集群端到端時間。
與之前一樣,基準(zhǔn)測試是在一臺 NVIDIA DGX-1 服務(wù)器上執(zhí)行的,該服務(wù)器有 8 個 V100 gpu 和兩個 20 核的 Xeon E5 – 2698 v4 cpu ,并進(jìn)行一輪訓(xùn)練、 shap 值計算和推理。此外,我們還共享了兩種內(nèi)存使用優(yōu)化,圖 4 描述了總體內(nèi)存使用比較。
圖 4 :內(nèi)存使用情況。
左兩列是 64 位數(shù)據(jù)類型訓(xùn)練的內(nèi)存使用情況,右兩列是 32 位數(shù)據(jù)類型訓(xùn)練的內(nèi)存使用情況。標(biāo)準(zhǔn)是指使用正常的數(shù)據(jù)矩陣和預(yù)測函數(shù)進(jìn)行訓(xùn)練。有效的方法是使用 DaskDeviceQuantileDMatrix 和 inplace_predict.
Scikit 學(xué)習(xí)包裝器
前面的章節(jié)考慮了“功能”接口的基本模型訓(xùn)練,但是,還有一個類似 scikit 學(xué)習(xí)估計器的接口。它更容易使用,但有更多的限制。在 XGBoost1 。 4 中,此接口與單節(jié)點實現(xiàn)具有相同的特性。用戶可以選擇不同的估計器,如 DaskXGBClassifier 用于分類,而 DaskXGBRanker 用于排名。查看參考資料以獲得可用估算器的完整列表: https://xgboost.readthedocs.io/en/latest/python/python_api.html#module-xgboost.dask 。
概括
我們已經(jīng)介紹了一個在 GPU 集群上使用 RAPIDS 庫加速 XGBoost 的示例,它顯示了使 XGBoost 代碼現(xiàn)代化可以幫助最大限度地提高培訓(xùn)效率。通過 XGBoost Dask 接口和 RAPIDS ,用戶可以通過一個易于使用的 API 實現(xiàn)顯著的加速。盡管 XGBoost-Dask 接口已經(jīng)達(dá)到了與單節(jié)點 API 的功能對等,但仍在繼續(xù)開發(fā),以便更好地與其他庫集成,實現(xiàn)超參數(shù)調(diào)優(yōu)等新功能。對于與 dask 接口相關(guān)的新功能請求,您可以在 XGBoost 的 GitHub 存儲庫 上打開一個問題。
關(guān)于作者
Jiaming Yuan 是 NVIDIA 的軟件工程師。
審核編輯:郭婷
-
筆記本電腦
+關(guān)注
關(guān)注
9文章
1414瀏覽量
48428 -
NVIDIA
+關(guān)注
關(guān)注
14文章
5052瀏覽量
103361 -
gpu
+關(guān)注
關(guān)注
28文章
4760瀏覽量
129134
發(fā)布評論請先 登錄
相關(guān)推薦
打開我的電腦網(wǎng)頁不是最大化新窗口
[轉(zhuǎn)]LabVIEW實現(xiàn)窗口最大化和最小化
labview能不能在運行的時候讓波形圖最大化
動態(tài)調(diào)用子程序窗口最大化問題
如何使應(yīng)用程序開機(jī)運行最大化
labview 編寫的應(yīng)用程序,怎么設(shè)置最大化后居中顯示
新的充電器拓?fù)?b class='flag-5'>最大化電池充電速度
如何在34970A上最大化測量速度?
Altium右上角沒有關(guān)閉和最大化的解決辦法
最大化電池操作時間
最大化自動化測試系統(tǒng)的精度
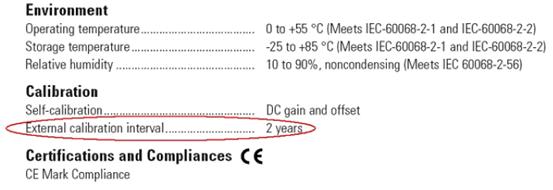
評論